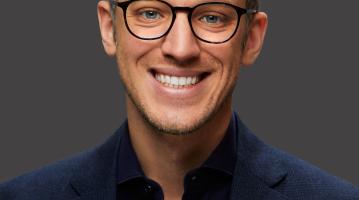
1.23.24-Japan-Ikigai-Labs

-
Video details
Generative AI for Tabular Data
-
Interactive transcript
STEPHANIE YOUNG: Hi, everyone. Thanks for having me. My name is Stephanie Young. I'm head of product at Ikigai Labs. Probably many of you are wondering how we picked the name Ikigai and if we're a Japanese company or not, and if we're not, how we ended up with the name Ikigai. So everyone I'm sure here knows about the Venn diagram and how ikigai means the purpose of life. Some people's ikigai is guitar or skiing or snowboarding or carbon capture. In our case, we decided that our ikigai is enterprise AI.
So our journey with MIT has been very long. So our founder, Devavrat Shah, has been teaching at MIT for 18 years in data science and AI. His first company, which is an AI company, he actually sold to Nike, and he's back for round two. He's very passionate about time series forecasting, which I'll explain more in a bit. We're now about 70 people based out of San Francisco and Cambridge.
So what is the problem that we're trying to solve here? So who in this room their companies have to predict into the future? So for many of your companies here, predicting into the future is at the core of everything your business does. So if you're manufacturing, you have to predict how much you need to manufacture. If you're doing retail or sales, you have to predict how much you're going to sell into the future.
That serves as a foundation or the backbone of how you manufacture and how you orchestrate the rest of your business. And every department from sales to finance to marketing to even product and engineering rely on an accurate prediction of the future in order to construct how they hire, how they resource, how they allocate resources, even raise money. So that's the problem that Ikigai, our Ikigai, is to solve.
So time series data, which essentially is forecasting and prediction data, is very complicated. It's a hard AI problem. And the classic example that people always give is the stock market. So why can't you predict things into the future? So for many of your businesses, the past is correlated with the future. And so we build AI models, generative models to solve that problem.
So time series data, you think about the core of it is your historical data. So if you're a retailer and you want to predict demand for a jacket or a pair of pants you're selling, you can look at how it's sold in the past. And the thing that Devavrat pioneered at Ikigai is the ability to bring in many other data sets and many other factors to improve that forecast.
So if you're selling a jacket and you're trying to predict it into the future, we can bring in things like there's a conference happening, the foot traffic at that retail mall, the weather, the temperature, even shipping container schedules, if a shipping container was delayed, it was a big storm on the Pacific. All these factors play a part in how that jacket sells into the future. And for the first time, we're able to bring all those factors in together in order to create an accurate crystal ball into the future for your business.
So think about us as a horizontal layer for forecasting. So forecasting happens in all different industries and all different use cases. So for example, a retailer. They might have to predict demand for a jacket or a pair of pants. But a supplier may need to predict pricing changes for vendor materials. You need to predict the price of ammonia or the price of denim, for example, or the price of corduroy. Hospitals. They might have to predict utility and occupancy. How many hospital beds are going to be taken up in a certain night.
Real estate. How can I predict how much foot traffic is going to enter my store or how many people are going to enter my mall? Sales. So your accounts. How can you predict which accounts are going to close and which accounts are going to fall through? Things like inventory. How can you predict stock outs and inventory shortages? And for banks, banking. How do you predict fraud? If someone's coming in, how can you predict that there's going to be a fraudulent transaction or a faulty charge?
So you can see that forecasting, even though it seems secondary or behind the scenes, is actually there and present in many of your business problems and present in almost every industry in every sector.
So I'll give an example here with a retailer. We work with a retailer in the US called Spirit Halloween. They are a pop up store for selling Halloween costumes, which is a big US holiday. They do 1 billion USD business in one month of October. And they have the hardest forecasting problem you can imagine. Because every single year, they have to introduce new costumes and they also retire costumes.
So at no point in time do they have accurate historical information to predict the future. And we heard from some Japanese companies that this is a similar problem that you have. You can change your configurations and work with small and sparse data sets so that at no point in time do you have enough historical data to accurately predict the future.
So what we do is that we run a process to reconcile, preprocess the data, and run it through our proprietary model, which we call AI Cast. That was developed in Devavrat's lab. And we use that along with many other factors and many other data sets to predict an accurate prediction of the future. And then we explain how that prediction came about. So if there's a spike in demand, we can explain this spike is because of last year's demand or it's because of a football game or it's because of XYZ reasons. So with Spirit, we worked with their data for a year and we ended up improving their forecast by 80%.
So why are we here and why Japan? So we mentioned before that forecasting is this hidden problem that's relevant in almost every business and almost every use case. We love to work in this intersection of many different hard problems. So manufacturing, supply chain, heavy chemicals, banking, some of these companies have the hardest forecasting problems and also such rich data to back up those forecasts. So this area is really rich. We have a lot of historical data and a lot of interesting ways to improve forecasting that ends up driving serious business value.
So the reason why we're here today is to meet all of you and also to posit that if you have an AI problem that you want to solve, if you have a forecasting or a problem that you think that we could use AI to solve, please come talk to us at the booth next door. The other thing is if you want to educate or train your members at your company about how to use AI within your corporation, we have an Ikigai Academy, which I'll show my email address after this if you want access to it. Essentially we created an academy that teaches enterprises how to use AI with tabular spreadsheet enterprise type data. Thank you.
-
Video details
Generative AI for Tabular Data
-
Interactive transcript
STEPHANIE YOUNG: Hi, everyone. Thanks for having me. My name is Stephanie Young. I'm head of product at Ikigai Labs. Probably many of you are wondering how we picked the name Ikigai and if we're a Japanese company or not, and if we're not, how we ended up with the name Ikigai. So everyone I'm sure here knows about the Venn diagram and how ikigai means the purpose of life. Some people's ikigai is guitar or skiing or snowboarding or carbon capture. In our case, we decided that our ikigai is enterprise AI.
So our journey with MIT has been very long. So our founder, Devavrat Shah, has been teaching at MIT for 18 years in data science and AI. His first company, which is an AI company, he actually sold to Nike, and he's back for round two. He's very passionate about time series forecasting, which I'll explain more in a bit. We're now about 70 people based out of San Francisco and Cambridge.
So what is the problem that we're trying to solve here? So who in this room their companies have to predict into the future? So for many of your companies here, predicting into the future is at the core of everything your business does. So if you're manufacturing, you have to predict how much you need to manufacture. If you're doing retail or sales, you have to predict how much you're going to sell into the future.
That serves as a foundation or the backbone of how you manufacture and how you orchestrate the rest of your business. And every department from sales to finance to marketing to even product and engineering rely on an accurate prediction of the future in order to construct how they hire, how they resource, how they allocate resources, even raise money. So that's the problem that Ikigai, our Ikigai, is to solve.
So time series data, which essentially is forecasting and prediction data, is very complicated. It's a hard AI problem. And the classic example that people always give is the stock market. So why can't you predict things into the future? So for many of your businesses, the past is correlated with the future. And so we build AI models, generative models to solve that problem.
So time series data, you think about the core of it is your historical data. So if you're a retailer and you want to predict demand for a jacket or a pair of pants you're selling, you can look at how it's sold in the past. And the thing that Devavrat pioneered at Ikigai is the ability to bring in many other data sets and many other factors to improve that forecast.
So if you're selling a jacket and you're trying to predict it into the future, we can bring in things like there's a conference happening, the foot traffic at that retail mall, the weather, the temperature, even shipping container schedules, if a shipping container was delayed, it was a big storm on the Pacific. All these factors play a part in how that jacket sells into the future. And for the first time, we're able to bring all those factors in together in order to create an accurate crystal ball into the future for your business.
So think about us as a horizontal layer for forecasting. So forecasting happens in all different industries and all different use cases. So for example, a retailer. They might have to predict demand for a jacket or a pair of pants. But a supplier may need to predict pricing changes for vendor materials. You need to predict the price of ammonia or the price of denim, for example, or the price of corduroy. Hospitals. They might have to predict utility and occupancy. How many hospital beds are going to be taken up in a certain night.
Real estate. How can I predict how much foot traffic is going to enter my store or how many people are going to enter my mall? Sales. So your accounts. How can you predict which accounts are going to close and which accounts are going to fall through? Things like inventory. How can you predict stock outs and inventory shortages? And for banks, banking. How do you predict fraud? If someone's coming in, how can you predict that there's going to be a fraudulent transaction or a faulty charge?
So you can see that forecasting, even though it seems secondary or behind the scenes, is actually there and present in many of your business problems and present in almost every industry in every sector.
So I'll give an example here with a retailer. We work with a retailer in the US called Spirit Halloween. They are a pop up store for selling Halloween costumes, which is a big US holiday. They do 1 billion USD business in one month of October. And they have the hardest forecasting problem you can imagine. Because every single year, they have to introduce new costumes and they also retire costumes.
So at no point in time do they have accurate historical information to predict the future. And we heard from some Japanese companies that this is a similar problem that you have. You can change your configurations and work with small and sparse data sets so that at no point in time do you have enough historical data to accurately predict the future.
So what we do is that we run a process to reconcile, preprocess the data, and run it through our proprietary model, which we call AI Cast. That was developed in Devavrat's lab. And we use that along with many other factors and many other data sets to predict an accurate prediction of the future. And then we explain how that prediction came about. So if there's a spike in demand, we can explain this spike is because of last year's demand or it's because of a football game or it's because of XYZ reasons. So with Spirit, we worked with their data for a year and we ended up improving their forecast by 80%.
So why are we here and why Japan? So we mentioned before that forecasting is this hidden problem that's relevant in almost every business and almost every use case. We love to work in this intersection of many different hard problems. So manufacturing, supply chain, heavy chemicals, banking, some of these companies have the hardest forecasting problems and also such rich data to back up those forecasts. So this area is really rich. We have a lot of historical data and a lot of interesting ways to improve forecasting that ends up driving serious business value.
So the reason why we're here today is to meet all of you and also to posit that if you have an AI problem that you want to solve, if you have a forecasting or a problem that you think that we could use AI to solve, please come talk to us at the booth next door. The other thing is if you want to educate or train your members at your company about how to use AI within your corporation, we have an Ikigai Academy, which I'll show my email address after this if you want access to it. Essentially we created an academy that teaches enterprises how to use AI with tabular spreadsheet enterprise type data. Thank you.