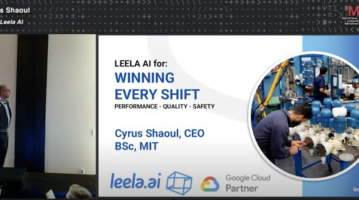
2024 MIT Houston Symposium: Startup Exchange - Prescient Devices

-
Interactive transcript
ANDY WANG: Thank you, everyone. It's a pleasure to be back in Houston, I think, for the sixth time in the past two months. So I'm here a lot. And some people even told me that I should find a place to live here because then I could reduce the carbon footprint that I generate through my trips.
So what we do is we build pretty large scale data solutions and AI solutions for customers in the energy space. And-- let's see how I use this. Is this just click? Yes!
So before I describe our products, I want to show a survey that was conducted at this year's SPE Advanced Technology conference from all the data professionals at the conference. And basically the question was, what was their biggest challenge in working with data and data science?
And the answer, as some of you probably know here, the biggest challenge is not building data science models. The biggest challenge is, first, just to get the data that you can use for data science. And this is followed by difficulty in scaling because performance is hard once you have large-scale solutions and lack of skills.
So we solve a lot of these problems for our customers. So essentially what we start with is we build these things called data pipelines. You may not be familiar with it, but it's basically real-time or historic data. Going through the data workflows, it gets cleaned, gets processed, and so that it's ready for AI to use. We can get process data from your rigs, from your production sites. And if you already have the raw data in your data warehouses or historians, we can grab those and clean that up.
And the next thing we do is we convert that data into something called events. So typical energy data, industrial data, there are time series data-- pressure, temperature. There's actually very little you can do with those data. But once you can convert them into events, either simple events like alarms or very complex events like component models, all of a sudden you can do very large scale-- BI analysis, AI, LLM, digital twins. So the conversion of events is actually very critical. And doing that at very large scale is difficult.
So if I ask all of you today that-- how do you build this type of data solutions? I think every company does this to some extent. I think the majority of you are going to say, we do it in software. Of course. That's how we know how to do this sort of things.
But all of us also know that software is super slow to build, requires very expensive software developers who do not have the domain expertise, and it's very inflexible to make any changes. So instead of doing things in software, we do it in graphical workflows. These are just drag and drop blocks, connect them by wires. And that's how we process data.
In this, graphical workflows can be deployed to different locations because your data is not necessarily all in the cloud. They are on your rigs, production sites. They can be in your private cloud. So there's little containerized data engines that can run in all different locations, collecting, processing all your data. Then we can fuse all of them together. And that's how we build solutions much, much faster and also simpler than software development. And I think, once you can do this, you can really accelerate how you can use the data in your organizations.
The other thing that we focus a lot is optimization of the compute architecture. So once you scale up, there's two bottlenecks you're going to face. One of them is performance, which keeps getting worse as you process more and more data. The other one is actually the compute cost. Everybody knows how expensive Azure and AWS are. So we have to really optimize at the CPU level in order to use every single CPU cycle so that we can scale the solutions very efficiently.
So let me give you an example of a solution. This is what we call operational digital twin solution for drilling rigs with our partner, Precision Drilling, one of the largest drilling contractors in North America. And what it does is basically monitor all the drilling equipment, like mud pumps, top drives, generators, and it uses a huge amount of data, billions of data points per day, tens of millions, 57 million database queries in order to generate those events. It's already deployed at over 100 rigs.
And who uses this, it turns out, is not just the field ops. When you have a really good digital twin solution, everyone uses it. And this is not the dream. This is actually how Precision uses today-- so from the field ops, the rig managers, to the remote ops team that use a lot of the automation feature in order to very quickly figure out what the problems are-- over 100 rigs, so they can only spend about two minutes per every rig to fix issues. So many, many of the things have to be automated.
And obviously, electrical engineers, they look at the data to understand what's going on, to data teams, generating BI reports, procurement CFO to look at budgeting, forecasting, and even the CEO gets a monthly report on what's going on with the rigs, how much spending are happening. So a good solution ties all the different units together.
This is something I want to show very quickly. This is how fast-- once you have this kind of graphical technology, how fast you can build solutions. The text is a little small there, but basically it took just two months for us to finish the digital twin solution for the mud pump and another month to iterate with two rig teams. You cannot do that in software ever.
And once the field teams tell us what they want, we get the solution implemented within that one month time, and then it starts to scale up. And you can see, we start to add AI models. We start to build digital twin for the other equipment. And in a year, it's over 50 rigs. In a year and a half, it's over 100 rigs. So this kind of development framework is super fast, and it gets a field to get engaged in a solution. If they're engaged, they're going to use it.
So this is just an example screen of a mud pump monitoring screen-- shows you what happens to the pump, what components are running close to the end of life, digital log app for them to put in what components are replaced, what issues they've seen. Those data actually goes back into training for the AI.
And there's also a very interesting AI behind the scenes. The field doesn't even know what technology is behind it. Why do they care? They just wanted to get their job done. But this AI predicts every single component lifetime issues from the day you put them in to the day of end of life. So it doesn't just say, oh, now is vibration. Something is going to happen.
But, instead, the first day you put it in, it's going to tell you what is going to happen through its life. And so we built these large solutions. We build them very quickly. And if you're interested, come to see our technology and our demo. Thank you.
[MUSIC PLAYING]
-
Interactive transcript
ANDY WANG: Thank you, everyone. It's a pleasure to be back in Houston, I think, for the sixth time in the past two months. So I'm here a lot. And some people even told me that I should find a place to live here because then I could reduce the carbon footprint that I generate through my trips.
So what we do is we build pretty large scale data solutions and AI solutions for customers in the energy space. And-- let's see how I use this. Is this just click? Yes!
So before I describe our products, I want to show a survey that was conducted at this year's SPE Advanced Technology conference from all the data professionals at the conference. And basically the question was, what was their biggest challenge in working with data and data science?
And the answer, as some of you probably know here, the biggest challenge is not building data science models. The biggest challenge is, first, just to get the data that you can use for data science. And this is followed by difficulty in scaling because performance is hard once you have large-scale solutions and lack of skills.
So we solve a lot of these problems for our customers. So essentially what we start with is we build these things called data pipelines. You may not be familiar with it, but it's basically real-time or historic data. Going through the data workflows, it gets cleaned, gets processed, and so that it's ready for AI to use. We can get process data from your rigs, from your production sites. And if you already have the raw data in your data warehouses or historians, we can grab those and clean that up.
And the next thing we do is we convert that data into something called events. So typical energy data, industrial data, there are time series data-- pressure, temperature. There's actually very little you can do with those data. But once you can convert them into events, either simple events like alarms or very complex events like component models, all of a sudden you can do very large scale-- BI analysis, AI, LLM, digital twins. So the conversion of events is actually very critical. And doing that at very large scale is difficult.
So if I ask all of you today that-- how do you build this type of data solutions? I think every company does this to some extent. I think the majority of you are going to say, we do it in software. Of course. That's how we know how to do this sort of things.
But all of us also know that software is super slow to build, requires very expensive software developers who do not have the domain expertise, and it's very inflexible to make any changes. So instead of doing things in software, we do it in graphical workflows. These are just drag and drop blocks, connect them by wires. And that's how we process data.
In this, graphical workflows can be deployed to different locations because your data is not necessarily all in the cloud. They are on your rigs, production sites. They can be in your private cloud. So there's little containerized data engines that can run in all different locations, collecting, processing all your data. Then we can fuse all of them together. And that's how we build solutions much, much faster and also simpler than software development. And I think, once you can do this, you can really accelerate how you can use the data in your organizations.
The other thing that we focus a lot is optimization of the compute architecture. So once you scale up, there's two bottlenecks you're going to face. One of them is performance, which keeps getting worse as you process more and more data. The other one is actually the compute cost. Everybody knows how expensive Azure and AWS are. So we have to really optimize at the CPU level in order to use every single CPU cycle so that we can scale the solutions very efficiently.
So let me give you an example of a solution. This is what we call operational digital twin solution for drilling rigs with our partner, Precision Drilling, one of the largest drilling contractors in North America. And what it does is basically monitor all the drilling equipment, like mud pumps, top drives, generators, and it uses a huge amount of data, billions of data points per day, tens of millions, 57 million database queries in order to generate those events. It's already deployed at over 100 rigs.
And who uses this, it turns out, is not just the field ops. When you have a really good digital twin solution, everyone uses it. And this is not the dream. This is actually how Precision uses today-- so from the field ops, the rig managers, to the remote ops team that use a lot of the automation feature in order to very quickly figure out what the problems are-- over 100 rigs, so they can only spend about two minutes per every rig to fix issues. So many, many of the things have to be automated.
And obviously, electrical engineers, they look at the data to understand what's going on, to data teams, generating BI reports, procurement CFO to look at budgeting, forecasting, and even the CEO gets a monthly report on what's going on with the rigs, how much spending are happening. So a good solution ties all the different units together.
This is something I want to show very quickly. This is how fast-- once you have this kind of graphical technology, how fast you can build solutions. The text is a little small there, but basically it took just two months for us to finish the digital twin solution for the mud pump and another month to iterate with two rig teams. You cannot do that in software ever.
And once the field teams tell us what they want, we get the solution implemented within that one month time, and then it starts to scale up. And you can see, we start to add AI models. We start to build digital twin for the other equipment. And in a year, it's over 50 rigs. In a year and a half, it's over 100 rigs. So this kind of development framework is super fast, and it gets a field to get engaged in a solution. If they're engaged, they're going to use it.
So this is just an example screen of a mud pump monitoring screen-- shows you what happens to the pump, what components are running close to the end of life, digital log app for them to put in what components are replaced, what issues they've seen. Those data actually goes back into training for the AI.
And there's also a very interesting AI behind the scenes. The field doesn't even know what technology is behind it. Why do they care? They just wanted to get their job done. But this AI predicts every single component lifetime issues from the day you put them in to the day of end of life. So it doesn't just say, oh, now is vibration. Something is going to happen.
But, instead, the first day you put it in, it's going to tell you what is going to happen through its life. And so we built these large solutions. We build them very quickly. And if you're interested, come to see our technology and our demo. Thank you.
[MUSIC PLAYING]