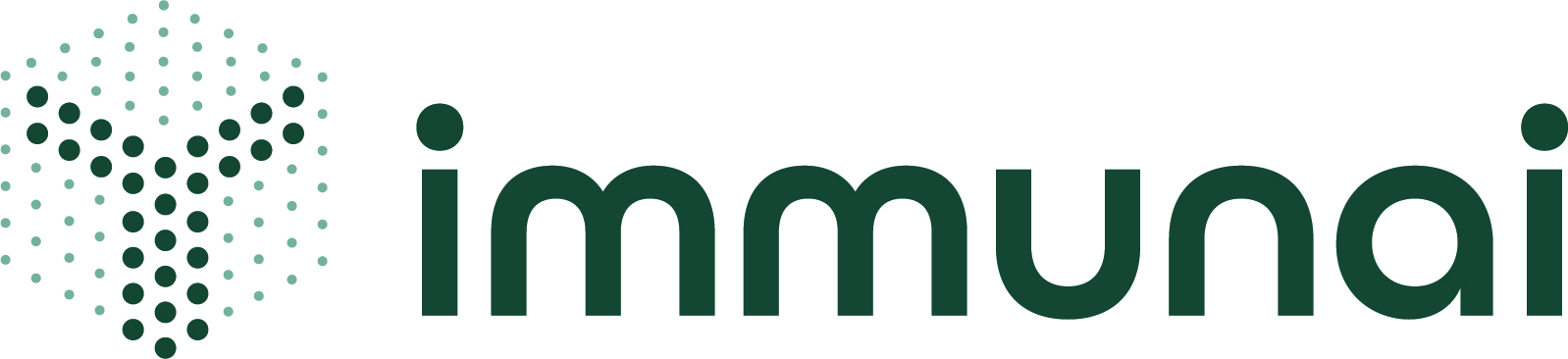
Immunai
Mapping the immune system to improve autoimmune and cancer therapies
Many of us have learned more about the human immune system over the past year than we have our entire lives. A similar awakening has occurred in the scientific community in recent years to decipher the immune system with the help of technologies such as single-cell multi-omic assays, big data tools, and machine learning (ML).
One of the leaders in unlocking the secrets of the immune system is a New York City-based startup called Immunai, which earlier this year announced $60 million in A round financing. The MIT STEX25 member’s co-founders and about 20 percent of the staff come from MIT.
Immunai has developed what it calls the world’s largest proprietary data set for clinical immunological data. The AMICA (Annotated Multi-omic Immune Cell Atlas) database of clinically annotated single-cell immune profiles draws on Immunai’s own single-cell multi-omics wet lab research, as well as data from research labs and hospitals around the world. Using big data and ML tools, the company mines AMICA for insights into the complex interactions of the immune system, from defense mechanisms for invading microbes to homegrown threats such as cancer and autoimmunity.
“The immune system is a highly complicated distributed system made up of trillions of immune cells distributed over different organs such as the lymph nodes, thymus, and spleen,” says Noam Solomon, Immunai CEO and cofounder.“Some have referred to the immune system as being infinitely complex. Yet, in recent years our understanding has expanded enormously. Immunai is one of the leaders in driving forward the industry’s understanding of the immune system ,” says Solomon.
The most important question for clinicians treating cancer patients is determining the right therapy. We can help them find the optimal therapy or combination therapy for a particular patient.
Immunai’s goal is to “fully map the immune system to reprogram immunity for better drug therapeutics development,” says Solomon, a former postdoctoral researcher at MIT’s Mathematics Department. “We are helping the immune system by making it more intelligent.”
More specifically, Immunai is providing clinicians with insights into selecting the right treatments for patients suffering from cancer and auto-immune diseases. Immunai’s platform also has potential for selecting treatments for viral infections, cardiovascular diseases, Alzheimers, diabetes, and “any other disease where inflammation plays a major role,” says Solomon. The company recently collaborated on a project to identify groups at risk for greater complications from Covid-19.
Since it is usually impossible to give patients more than one treatment at a time, clinicians need help in choosing from a growing arsenal of therapies that include recently approved immunotherapies such as Anti-CTLA and Anti-PD-1. “The most important question for clinicians treating cancer patients is determining the right therapy,” says Solomon. “We can help them find the optimal therapy or combination therapy for a particular patient.”
Immunai measures the immune profile of cancer patients at different time points, pre and post-treatment, as well as the immune response and inflammation processes. It then correlates the findings to the clinical response.
“Different patients sometimes have similar immune profiles, which enables us to tease out the signals that are later correlated to the clinical response,” says Solomon. “Over time we have found some important therapeutic and diagnostic signals. With cancer patients, we can measure how they respond to or resist response to different therapies.”
Another major challenge for clinicians is determining whether a drug is working. “Even a few months after therapy it is not always easy to judge success,” says Solomon. “The clinician also needs to know whether the patient will relapse. Ideally, one could predict whether the patient would have a toxic event prior to therapy. Our platform can help tease out all these questions.”
Single cell multi-omics
Immunai has embraced the increasingly popular approach of measuring tissue on a cell-by cell basis instead of bulk assays. Studying cells individually makes it easier to identify rare cell types and offers a finer-grained understanding of cellular function.
The single-cell assays are measured with multiple modalities -- integrating many different omics including genomics, single-cell RNA, proteome data, and other assays. “Each of these assays provides a different perspective on the cell,” says Solomon. “By measuring more and more omics over tens of thousands of different cells, we can assemble a full profile of a patient’s immune system.”
The vertically integrated platform starts with pre-processing, processing, and sequencing samples in the company’s wet lab in New York. The resulting data is uploaded to their cloud platform where Immunai researchers in New York, San Francisco, and Tel Aviv integrate the data with batches gathered from around the world.
“We have data from samples that were stored in different environmental conditions coming from different institutions and countries, which results in technical noise,” says Solomon. “Our first task is to harmonize the data as if all the samples came from a single hospital.”
Immunai combines big data techniques with ML to process “terabytes of information using very complicated ML models,” says Solomon. “Big data is the fuel and the AI, ML, and the engineering pipeline are the engine. Different types of ML models are used to mine insights that explain the mechanisms for resistance and action for a given drug, including models that predict which patient will respond to which therapy.”
The company’s functional genomics platform validates correlations in independent cohorts that are then “siloed out of the database,” says Solomon. “The next level is doing individual and in vivo validation. With our CRISPR editing platform, we can test hypotheses and validate them within cell lines and in animal models.”
Immunai works in partnerships with leading academic institutions, hospitals, and Fortune 100 biotech and biopharma companies to develop customized studies. “We are answering questions like how the mechanism of action and resistance work for a particular therapy. With cancer, we can determine whether to use a particular immunotherapy and whether to use it alone or in conjunction with chemotherapy or other therapies.”
Fine-tuning clinical trials and discovering novel targets
Some of Immunai’s clients use the platform to enhance the design of clinical trials. “It is difficult to decide on the inclusion and exclusion criteria for clinical trialsand how to improve patient segmentation criteria,” says Solomon. “To address these questions, we train our ML models on different indications and therapies from the database so they can determine the probability that patient A will respond to therapy B.”
The platform can also help determine the right dose or dose escalation for a particular patient in a trial. “We can determine if a patient will be a super non-responder or who will have severe adverse responses.”
We can look for a molecule to target this gene and knock it out or promote downregulation of the gene in a specific cell. Understanding the resistance mechanisms for drugs can lead to better diagnostics, as well as better therapeutics and drug discovery.
A similar, albeit more sophisticated, process can be used for novel drug discovery. “By answering these questions, we essentially identify immunological nodal arrangements and pathways for new targets,” says Solomon. “Say you could identify that a specific T-cell subpopulation is correlated with resistance to a specific therapy. Perhaps you could see that a specific gene is related to that and that if the gene is upregulated, the patient will have a severe event. We can look for a molecule to target this gene and knock it out or promote downregulation of the gene in a specific cell. Understanding the resistance mechanisms for drugs can lead to better diagnostics, as well as better therapeutics and drug discovery.”
Insights gleaned from the AMICA database have furthered fundamental research into the immune system. “When we measure the immune systems as a holistic system, we are able to find both similarities and differences,” says Solomon. “We have discovered some interesting relationships between autoimmunity and cancer. Inflammation is the thing that ties them all together. We have also identified some commonalities to the behavior of more effective T-cells.”
Moving toward in-house therapy development
The genesis of Immunai started several years ago with discussions between Solomon and Luis Voloch, an ML expert who had been working at Palantir after leaving MIT. “We both believed that by building the right database for the immune system we could find some interesting diagnostic and therapeutic insights,” says Solomon.
As the co-founders began formulating ideas for a startup, they set about strengthening their medical and biological expertise. They brought in Ansuman Satpathy, an assistant professor of immunology and pathology at Stanford, as well as Dan Littman, an HHMI investigator, NYU professor, and world famous immunologist. . The third key addition was Dr. Danny Wells,, an expert in cancer immunotherapy who left the Parker Institute to join Immunai as SVP of Strategic Research. Like Littman and Satpathy, Wells is a Scientific Co-founder at Immunai. Other staff includes a mix of computer scientists from Google, Facebook, and other tech firms along with computational biologists, immunologists, genomics experts and others.
After the launch in 2019, Immunai exited stealth mode in May 2020 to announce a $20 million round of seed funding. Immunai also joined MIT’s Startup Exchange, STEX25. “STEX25 is a great way to be connected to the cutting edge of science and technology,” says Solomon. “You get visibility to leaders in the industry and interact with people who are experiencing the same process.”
Later in the year, Immunai announced a collaboration with Baylor College of Medicine to assist in developing novel CAR-NKT therapies. “We already have promising findings for the next-gen CAR-NKT therapies,” says Solomon.
With the new $60M round, Immunai will continue to build out Immunai’s functional genomics platform. “Our goal is to streamline the in vivo assay process to validate 10 times faster than any existing proof.”
The next step in Immunai’s journey is to develop an in-house therapeutics program. In the meantime, there are plenty of problems to be solved in answering complex client questions. “We will continue to collect, integrate, and curate the world’s largest immunological database to answer the most important and complicated questions in clinical target design and novel target discovery and validation,” says Solomon.