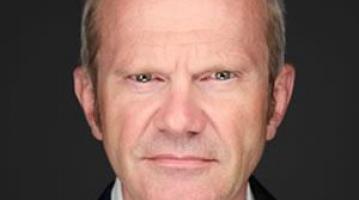
11.15-16.23-RD-Kebotix
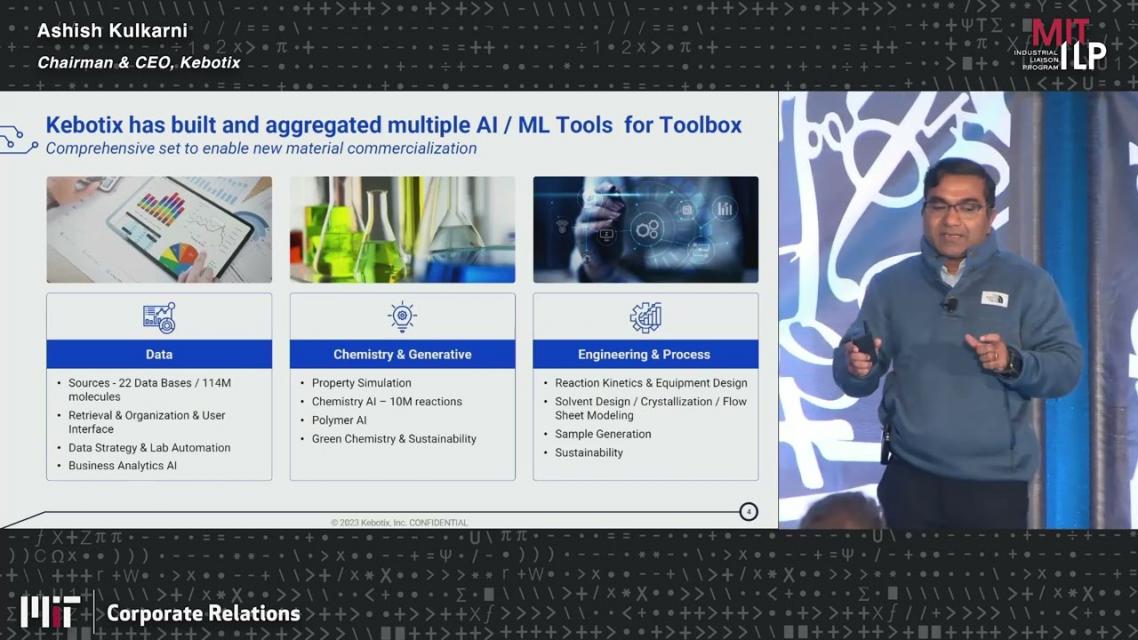
-
Video details
Transforming Materials Innovation
-
Interactive transcript
ASHISH KULKARNI: All right, I'm here to talk about how to use AI/ML to accelerate R&D in the world of materials. We are related to MIT. One of our co-founders, who is CTO, is MIT alum, and I come to this stage after 25 years of industrial R&D and all the frustrations associated with that. So hope is AI/ML can change the way R&D is done in the materials world.
If you think about this, on the left-hand side is some variant of stage gate process. How many of you are here from industry? And how many of you are used to stage gate process? How many of you are happy with it? OK. I rest my case.
Everybody uses some variant of this, and 90% of the people who follow this process are working very hard to work around this process, right? And this is the state of affairs inside the industry. The reason for that is it is slow, it is siloed, lower success rate, talent draws, organizational boundaries, and it is very frustrating for most of the CXOs.
I was CTO for 10 years at three large chemical companies, and I can vouch for that. And it needs a solution. Because the world does need a new way of thinking about new materials. It's very important for ESG reasons.
Imagine a world where you can do discovery, manufacturing, and commercialization of new materials, all in an interconnected way. Where on the left-hand side, you have a toolbox of different AI agents or apps, and on the right-hand side is an AI-enabled gated process, but not gated for a functional or a de-risking reason, but you are gated because you want to break your launches into multiple smaller launches where you become successively faster.
When you use these kind of tools and you change the materials from molecules to applications, but to properties and spaces, you can dramatically change the way innovation in materials is done. You can do it simultaneously. You can have enormous amount of speed. You can actually do it at scale.
You are learning and innovation happens at the same time. It doesn't happen to happen in isolation. And you can do multiple launches in the same amount of time. Every progressive launch becomes very effective. It becomes faster. Your generative phase gives you ideas. Your discriminative phase allows you to de-risk very quickly, and you can have a smaller launch.
That's the model Kebotix is building. We have a few of the apps that you see on the left-hand side already applied. We have done quite a few practical problems related to industry, because I have seen those problems. If you think about in any industrial setup, there are methods that R&D follows. There are manufacturing methods. There are supply chain challenges, trying to find suppliers, formulation methods, and regulatory challenges, depending on which industry you are selling your material into.
But AI/ML tools exist across the board. There are some tools that Kebotix has developed on our own. There are some tools we have aggregated from other partners. And our goal is to integrate them seamlessly so customers can launch their new projects and new products rapidly.
Complementing that, we have also thought about algorithms and numerical methods that combine properties and spaces in terms of data. Everybody thinks you need reams and reams of data. We have thought about how to do it with less data.
We have generative algorithms. But more importantly, we have also complemented with engineering and process variables which allows you to scale the chemistry in a way where AI/ML models can be very practical. To prove my point, we have done about 20 to 25 customer paid projects. We have been able to deliver over 250 plus molecules on an average cycle at time of two to three months, 13 different classes at a speed that is 10x faster than the traditional approach.
You can see, on the right-hand side, almost all projects have led to either a Kebotix patent or a customer patent is how we have done the work. Here is a very specific example where you will notice a customer wanted a new pigment for a polymer called nylon for an automotive applications to be able to recycle. You will see on the left hand side a traditional approach.
Specs were given. But more importantly, on the right-hand side, we combined AI/ML approaches, experimental approach, and expert, and made it all happen in less than six months from a problem to a sample. And that's what I see the world of materials R&D. I think the world needs it, where you can combine everything into one big app store set of agents where you can do lab work, manufacturing, and commercialization, all at the same time.
What we are looking for is we are looking for people who can work with us. If you have rapid R&D challenges, where you are struggling with your traditional stage gate process, we would love to partner with you. Though we have focused on three very distinct areas of green cement, green polymers, and generic small molecule pharma, because of the chemistry reasons, it can be applied to a wide variety of-- wide variety of applications. Any challenges you have, would be more than happy to chat with you in the other hall.
Thank you so much for the opportunity. Thank you MIT. Look forward to talking to you guys.
-
Video details
Transforming Materials Innovation
-
Interactive transcript
ASHISH KULKARNI: All right, I'm here to talk about how to use AI/ML to accelerate R&D in the world of materials. We are related to MIT. One of our co-founders, who is CTO, is MIT alum, and I come to this stage after 25 years of industrial R&D and all the frustrations associated with that. So hope is AI/ML can change the way R&D is done in the materials world.
If you think about this, on the left-hand side is some variant of stage gate process. How many of you are here from industry? And how many of you are used to stage gate process? How many of you are happy with it? OK. I rest my case.
Everybody uses some variant of this, and 90% of the people who follow this process are working very hard to work around this process, right? And this is the state of affairs inside the industry. The reason for that is it is slow, it is siloed, lower success rate, talent draws, organizational boundaries, and it is very frustrating for most of the CXOs.
I was CTO for 10 years at three large chemical companies, and I can vouch for that. And it needs a solution. Because the world does need a new way of thinking about new materials. It's very important for ESG reasons.
Imagine a world where you can do discovery, manufacturing, and commercialization of new materials, all in an interconnected way. Where on the left-hand side, you have a toolbox of different AI agents or apps, and on the right-hand side is an AI-enabled gated process, but not gated for a functional or a de-risking reason, but you are gated because you want to break your launches into multiple smaller launches where you become successively faster.
When you use these kind of tools and you change the materials from molecules to applications, but to properties and spaces, you can dramatically change the way innovation in materials is done. You can do it simultaneously. You can have enormous amount of speed. You can actually do it at scale.
You are learning and innovation happens at the same time. It doesn't happen to happen in isolation. And you can do multiple launches in the same amount of time. Every progressive launch becomes very effective. It becomes faster. Your generative phase gives you ideas. Your discriminative phase allows you to de-risk very quickly, and you can have a smaller launch.
That's the model Kebotix is building. We have a few of the apps that you see on the left-hand side already applied. We have done quite a few practical problems related to industry, because I have seen those problems. If you think about in any industrial setup, there are methods that R&D follows. There are manufacturing methods. There are supply chain challenges, trying to find suppliers, formulation methods, and regulatory challenges, depending on which industry you are selling your material into.
But AI/ML tools exist across the board. There are some tools that Kebotix has developed on our own. There are some tools we have aggregated from other partners. And our goal is to integrate them seamlessly so customers can launch their new projects and new products rapidly.
Complementing that, we have also thought about algorithms and numerical methods that combine properties and spaces in terms of data. Everybody thinks you need reams and reams of data. We have thought about how to do it with less data.
We have generative algorithms. But more importantly, we have also complemented with engineering and process variables which allows you to scale the chemistry in a way where AI/ML models can be very practical. To prove my point, we have done about 20 to 25 customer paid projects. We have been able to deliver over 250 plus molecules on an average cycle at time of two to three months, 13 different classes at a speed that is 10x faster than the traditional approach.
You can see, on the right-hand side, almost all projects have led to either a Kebotix patent or a customer patent is how we have done the work. Here is a very specific example where you will notice a customer wanted a new pigment for a polymer called nylon for an automotive applications to be able to recycle. You will see on the left hand side a traditional approach.
Specs were given. But more importantly, on the right-hand side, we combined AI/ML approaches, experimental approach, and expert, and made it all happen in less than six months from a problem to a sample. And that's what I see the world of materials R&D. I think the world needs it, where you can combine everything into one big app store set of agents where you can do lab work, manufacturing, and commercialization, all at the same time.
What we are looking for is we are looking for people who can work with us. If you have rapid R&D challenges, where you are struggling with your traditional stage gate process, we would love to partner with you. Though we have focused on three very distinct areas of green cement, green polymers, and generic small molecule pharma, because of the chemistry reasons, it can be applied to a wide variety of-- wide variety of applications. Any challenges you have, would be more than happy to chat with you in the other hall.
Thank you so much for the opportunity. Thank you MIT. Look forward to talking to you guys.