Covariance.ai: The World Your CEO Can’t See
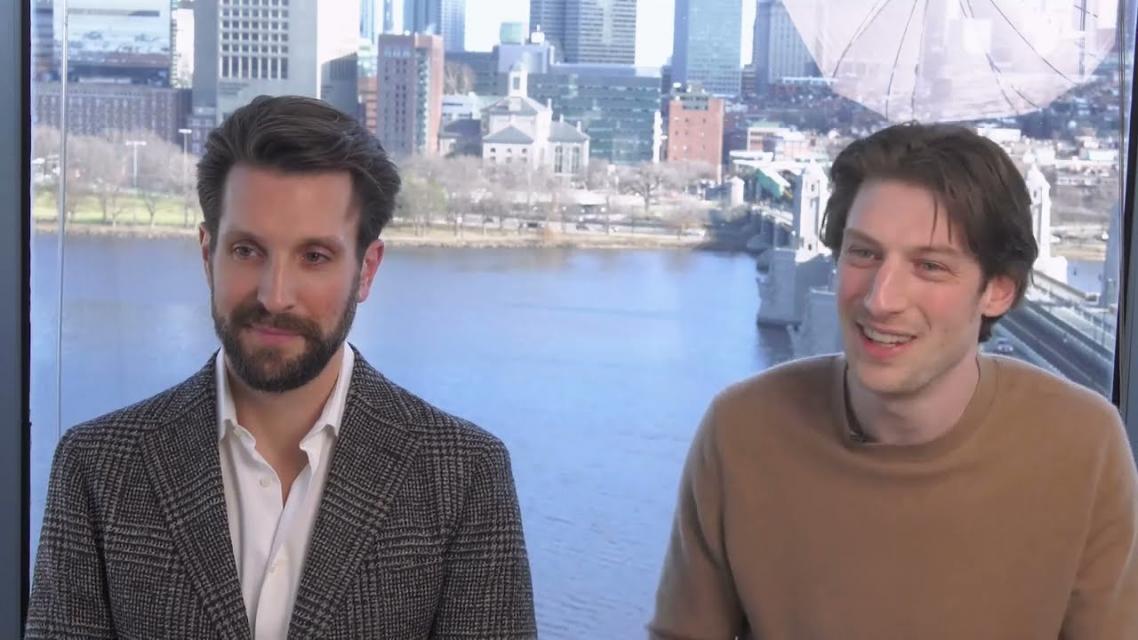
-
Interactive transcript
[MUSIC PLAYING]
MICHAEL FLEDER: Mike Fleder, CEO, founder of Covariance. This is Matt, our president and co-founder. We started at MIT. We do one thing. We predict corporate financials from external data. We do this through new theory that we developed here and are now successfully commercializing.
So I've spent time in quantitative finance, in addition to academic research in machine learning. And in seeing both, the inside the lab and outside the lab, I saw that a key problem showing up in every industry, Wall Street and elsewhere, was people are trying to track companies. What were Chipotle sales yesterday?
It's very hard to answer that question. And the best companies in answering that question have been a small number of hedge funds. The tools to answer that question, like what were sales yesterday at a retailer, really don't exist in the market today.
And part of that is the theory has been missing. In the last 20 years of machine learning, folks have focused on big data, large piles of data, answering questions like, is this a cat or not. And this is really a small data problem. There might be lots and lots of data, but ground truth, like sales information, shows up rarely and infrequently and coarsely. In a quarterly report, sales for the last 90 days are reported with lag.
So I want to know what happened yesterday at a store. And to do that, we need to combine tons of data, like tons of noisy, biased proxy signals, with very limited ground truth. And the math to do that started with my PhD. We're now successfully commercializing that, and as a result have had inbound from every industry, hedge funds, Wall Street, and corporates now as well.
MATT PERLEIN: Your competition and your market are changing at an accelerating pace. Companies are making multibillion dollar decisions with only a fuzzy view of what's happening outside their four walls. Oftentimes, they're looking at lots of different conflicting reports. They're looking at very delayed or lagged pictures of the outside world.
Nothing adds up to their internal numbers. And a lot of the really detailed KPIs that you need are missing. So what happens is you have low confidence in decisions. With multiple views you're not able to act as quickly or as decisively as you need to in a very quickly changing market.
Covariance provides a unified real-time picture of what's happening with your competitors, your market, and your customers.
MICHAEL FLEDER: We started here at MIT in LIDS with Professor Devavrat Shah, with whom I did my dissertation. From the work we did together we put out papers, a book chapter, and some news articles as well. And from that work, we received a ton of inbound from different industries and asset managers, corporates, data vendors, all asking about the same thing, which is how do you take these piles of external data, tiny bits of ground truth, and combine those together to answer simple questions like what were sales yesterday at Chipotle? Or did someone buy a burrito or not?
And it turns out, that worked really well. From the work we put out beat one of the hardest forecasting benchmarks in the world, on Wall Street. And as a result, we said, hey, here's a competitive edge that's useful not just for asset managers, but for corporates as well, who just don't have these tools today. And so the thesis has been there's interesting opportunities across verticals.
And that inbound has kept us busy ever since. So we have actually really done no marketing and very little outbound. And we've just mostly been responding to inbound to date. And what we're doing is we're now operating at petabyte scale, processing tons of external and even internal data to give our customers an outsize edge in these small poker games that they're engaged in with competitors.
MATT PERLEIN: Our early customers are companies in highly competitive, small poker games, and also companies that are in industries that are in flux. It could be a new market entrant. It could be changing competitive dynamics, or any other structural change. In both cases there's outsized value in having a real-time hyper-granular view of what's happening with your competitors in your market.
MICHAEL FLEDER: Common solutions to competitive customer intelligence is to buy tons and tons of reports. And you get market reports, which are bought, you know, like aggregated or delayed and often coarse and biased. You might get disclosures for public companies, but they're delayed, and also rolled up to 90 days or more for international firms.
There does not exist a system, or before us, theory, to combine all of these types of data together into one view. Customers come to us for confidence. Tell me what's happening outside my walls. And to do that requires combining every source of information into one framework, in a precise, quantitative, statistically rigorous manner, to answer a very simple question, what's going on across the street? What's going on when my customer is leaving the store?
MATT PERLEIN: We've gotten inbound from every industry and the solution applies, because this type of external data exists for every space. Where we're starting is with enterprises, very large enterprises in the B2C or B2B2C space, anything that ultimately touches the consumer. And we provide next generation, competitive market, and customer intelligence.
We're looking for partners who are very vision-aligned to what we want to build and want to democratize this through not just a small strategy team, but also operationally throughout their organization. We feel like we can iterate and evolve the platform fastest if it's well-used. So we want customers who are excited to bring this to more of their company.
We're looking for partners who don't just want to use this platform as a nice-to-have, and to inform quarterly or annual strategy. What we want is partners who are excited to use this in day-to-day, week-to-week, month-to-month operations, and integrate it very tightly into their operational management of their company.
MICHAEL FLEDER: The work product is really simple, you know, what's happening outside my walls. The steps to do that are unbelievably complicated. And there are a number of innovations that were required. So I see there are innovations from machine learning theory to practice. But then there's also innovations in setting up a business properly and running it.
I really liked MIT Professor Andrew Lo was talking about a famous hedge fund manager and described that a lot of the magic of that success at a particular firm was not just in any particular modeling technique, but also in how do you set up the business as this effectively forecasting factory. And we've really spent an enormous amount of effort to do that at Covariance, where we take just unbelievably incredible care to make sure that everything is processed in a tightly controlled fashion, which requires innovations to set up effectively that signal processing assembly line.
In addition to the innovations that are required, it's sort of each step in the factory. So there might be very novel work that's happening in, you know, culled like a workstation in a factory might come from a paper, might come from something new that we've invented today or yesterday. But then that combination of the innovations both on the business side, but also and the technical side, we see as just fundamental requirements for getting this right.
And ultimately that's a lot of very complicated-- It's a lot of complicated stuff. But the answer is someone bought a burrito yesterday. Anyone can understand. So we see innovation get everything together, but make it really with the end goal of making something that's easy to understand and use for our customers.
MATT PERLEIN: Ultimately scale for us is about access. Historically, there were folks that were focused on this problem. But they were at a handful of hedge funds. And these sorts of techniques were not accessible to the broader market. For us, it's a democratization play, right?
The way that we've set up our technology, the factory that Mike talks about, is designed so that we can do this for more and more companies and more and more spaces. We feel that the more people have access to this type of technology, the better decisions that they can make for their suppliers, for their employees, and for their customers.
-
Interactive transcript
[MUSIC PLAYING]
MICHAEL FLEDER: Mike Fleder, CEO, founder of Covariance. This is Matt, our president and co-founder. We started at MIT. We do one thing. We predict corporate financials from external data. We do this through new theory that we developed here and are now successfully commercializing.
So I've spent time in quantitative finance, in addition to academic research in machine learning. And in seeing both, the inside the lab and outside the lab, I saw that a key problem showing up in every industry, Wall Street and elsewhere, was people are trying to track companies. What were Chipotle sales yesterday?
It's very hard to answer that question. And the best companies in answering that question have been a small number of hedge funds. The tools to answer that question, like what were sales yesterday at a retailer, really don't exist in the market today.
And part of that is the theory has been missing. In the last 20 years of machine learning, folks have focused on big data, large piles of data, answering questions like, is this a cat or not. And this is really a small data problem. There might be lots and lots of data, but ground truth, like sales information, shows up rarely and infrequently and coarsely. In a quarterly report, sales for the last 90 days are reported with lag.
So I want to know what happened yesterday at a store. And to do that, we need to combine tons of data, like tons of noisy, biased proxy signals, with very limited ground truth. And the math to do that started with my PhD. We're now successfully commercializing that, and as a result have had inbound from every industry, hedge funds, Wall Street, and corporates now as well.
MATT PERLEIN: Your competition and your market are changing at an accelerating pace. Companies are making multibillion dollar decisions with only a fuzzy view of what's happening outside their four walls. Oftentimes, they're looking at lots of different conflicting reports. They're looking at very delayed or lagged pictures of the outside world.
Nothing adds up to their internal numbers. And a lot of the really detailed KPIs that you need are missing. So what happens is you have low confidence in decisions. With multiple views you're not able to act as quickly or as decisively as you need to in a very quickly changing market.
Covariance provides a unified real-time picture of what's happening with your competitors, your market, and your customers.
MICHAEL FLEDER: We started here at MIT in LIDS with Professor Devavrat Shah, with whom I did my dissertation. From the work we did together we put out papers, a book chapter, and some news articles as well. And from that work, we received a ton of inbound from different industries and asset managers, corporates, data vendors, all asking about the same thing, which is how do you take these piles of external data, tiny bits of ground truth, and combine those together to answer simple questions like what were sales yesterday at Chipotle? Or did someone buy a burrito or not?
And it turns out, that worked really well. From the work we put out beat one of the hardest forecasting benchmarks in the world, on Wall Street. And as a result, we said, hey, here's a competitive edge that's useful not just for asset managers, but for corporates as well, who just don't have these tools today. And so the thesis has been there's interesting opportunities across verticals.
And that inbound has kept us busy ever since. So we have actually really done no marketing and very little outbound. And we've just mostly been responding to inbound to date. And what we're doing is we're now operating at petabyte scale, processing tons of external and even internal data to give our customers an outsize edge in these small poker games that they're engaged in with competitors.
MATT PERLEIN: Our early customers are companies in highly competitive, small poker games, and also companies that are in industries that are in flux. It could be a new market entrant. It could be changing competitive dynamics, or any other structural change. In both cases there's outsized value in having a real-time hyper-granular view of what's happening with your competitors in your market.
MICHAEL FLEDER: Common solutions to competitive customer intelligence is to buy tons and tons of reports. And you get market reports, which are bought, you know, like aggregated or delayed and often coarse and biased. You might get disclosures for public companies, but they're delayed, and also rolled up to 90 days or more for international firms.
There does not exist a system, or before us, theory, to combine all of these types of data together into one view. Customers come to us for confidence. Tell me what's happening outside my walls. And to do that requires combining every source of information into one framework, in a precise, quantitative, statistically rigorous manner, to answer a very simple question, what's going on across the street? What's going on when my customer is leaving the store?
MATT PERLEIN: We've gotten inbound from every industry and the solution applies, because this type of external data exists for every space. Where we're starting is with enterprises, very large enterprises in the B2C or B2B2C space, anything that ultimately touches the consumer. And we provide next generation, competitive market, and customer intelligence.
We're looking for partners who are very vision-aligned to what we want to build and want to democratize this through not just a small strategy team, but also operationally throughout their organization. We feel like we can iterate and evolve the platform fastest if it's well-used. So we want customers who are excited to bring this to more of their company.
We're looking for partners who don't just want to use this platform as a nice-to-have, and to inform quarterly or annual strategy. What we want is partners who are excited to use this in day-to-day, week-to-week, month-to-month operations, and integrate it very tightly into their operational management of their company.
MICHAEL FLEDER: The work product is really simple, you know, what's happening outside my walls. The steps to do that are unbelievably complicated. And there are a number of innovations that were required. So I see there are innovations from machine learning theory to practice. But then there's also innovations in setting up a business properly and running it.
I really liked MIT Professor Andrew Lo was talking about a famous hedge fund manager and described that a lot of the magic of that success at a particular firm was not just in any particular modeling technique, but also in how do you set up the business as this effectively forecasting factory. And we've really spent an enormous amount of effort to do that at Covariance, where we take just unbelievably incredible care to make sure that everything is processed in a tightly controlled fashion, which requires innovations to set up effectively that signal processing assembly line.
In addition to the innovations that are required, it's sort of each step in the factory. So there might be very novel work that's happening in, you know, culled like a workstation in a factory might come from a paper, might come from something new that we've invented today or yesterday. But then that combination of the innovations both on the business side, but also and the technical side, we see as just fundamental requirements for getting this right.
And ultimately that's a lot of very complicated-- It's a lot of complicated stuff. But the answer is someone bought a burrito yesterday. Anyone can understand. So we see innovation get everything together, but make it really with the end goal of making something that's easy to understand and use for our customers.
MATT PERLEIN: Ultimately scale for us is about access. Historically, there were folks that were focused on this problem. But they were at a handful of hedge funds. And these sorts of techniques were not accessible to the broader market. For us, it's a democratization play, right?
The way that we've set up our technology, the factory that Mike talks about, is designed so that we can do this for more and more companies and more and more spaces. We feel that the more people have access to this type of technology, the better decisions that they can make for their suppliers, for their employees, and for their customers.