Gamalon
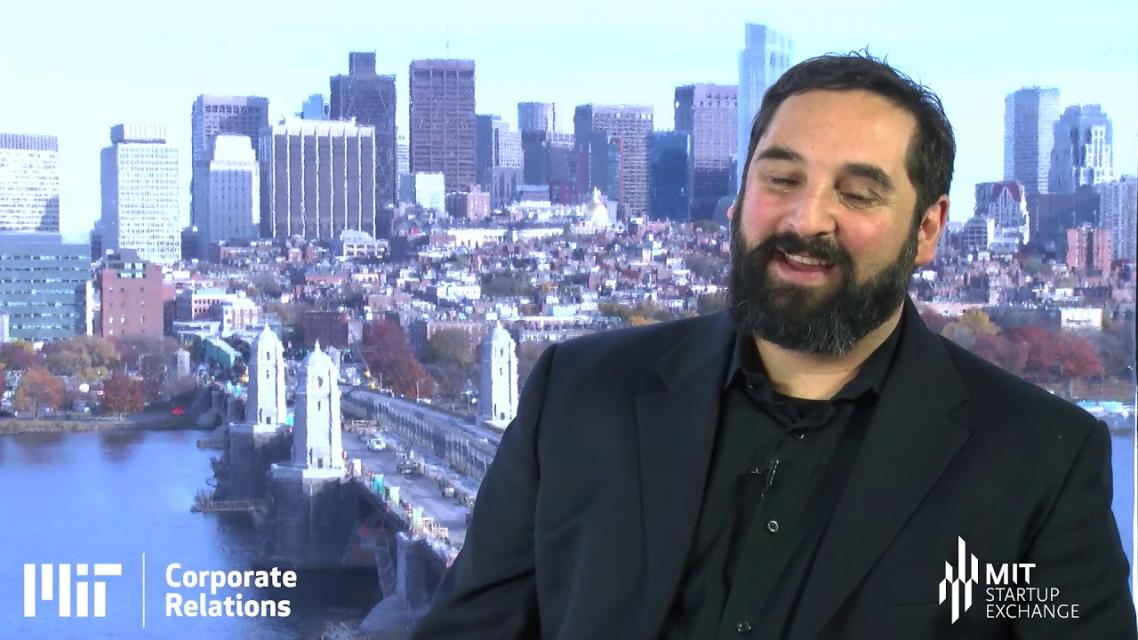
-
Interactive transcript
BEN VIGODA: My name is Ben Vigoda. I'm the CEO of Gamalon. And we are developing the next generation in deep learning and machine learning. And we're applying that to bringing data together, essentially automatically and autonomously processing data from many different sources and joining it.
So my experience at MIT, getting my PhD at the MIT Media Lab with Professor Neil Gershenfeld-- we built the first probability-based microchips. And they're really the first microprocessor architecture specifically designed to perform machine learning algorithms or applications. And that grew up into my first startup foray, which was called Lyric Semiconductor. And we eventually grew up and joined a larger organization, Analog Devices, and put the chips into all kinds of everyday objects and products which probably everyone uses-- phones, and cell phone base stations, and cars, and so forth.
And one of the things I learned from that experience was that hardware and software go hand-in-hand together. Venturing out to start my second company, Gamalon, was really going back to software from hardware, going back to the algorithms and saying, now that we've seen how these initial algorithms compile onto hardware, can we re-envision the design flow and the tool chain for implementing statistical machine learning and deep learning?
Gamalon has-- currently, we're not using any hardware or special hardware. We use the cloud. And we're actually portable across all of the different public clouds. So we run on Google and Microsoft and Amazon clouds, and so forth. And there's a lot in common between the parallelism that happens inside of a specially designed chip and the parallelism and kinds of communication patterns and so forth and compromises that you have to make when you have lots of machines in a data center, in the cloud. So there's many interesting technological relations there. But Gamalon is a cloud SAS company.
And two things about that-- one of the things we do is we-- basically, people can upload database extracts, CSV files, Excel spreadsheets, and so forth, and just dump them all up onto this web page. And our machine will read through it all, and it'll give you back a single, unified, perfect spreadsheet with-- if there was one spreadsheet that initially had some information about a person, and maybe where they lived, and another one had, maybe, an address, and-- I don't know-- something else, like the cost of their home, then you could join those three things together now, even if they were written differently, so even if they were written in colloquial human language, not necessarily written out in machine code.
And what we're really talking about with this new kind of machine learning is a way of bringing stories into deep learning. So it's what we call prior knowledge. So today, the state-of-the-art machine learning doesn't know anything until it meets the data. So it's a blank algorithm. It's essentially a tabula rasa. It has no prior, almost no prior.
And you give it some data, and it learns from that data. It sort of shapes itself. But before you give it some data, the same algorithm could essentially run on audio, video, texts, very similar. Whereas human thought, probably-- and we think the future of machine learning-- lies in the machine actually knowing some stuff. And so we call those stories.
And our machine learns stories from data, but then they're articulated in the system. So we can go in and read the stories it's learned. They're written in Python. And we can see what it's thinking. And when it goes and applies the stories it's learned to the future work that it does, you can have a good sense-- like a human expert with a certain background, you can have a pretty good sense of what kinds of background or prior leanings or ideas it's going to bring to examining a new piece of data.
[MUSIC PLAYING]
-
Interactive transcript
BEN VIGODA: My name is Ben Vigoda. I'm the CEO of Gamalon. And we are developing the next generation in deep learning and machine learning. And we're applying that to bringing data together, essentially automatically and autonomously processing data from many different sources and joining it.
So my experience at MIT, getting my PhD at the MIT Media Lab with Professor Neil Gershenfeld-- we built the first probability-based microchips. And they're really the first microprocessor architecture specifically designed to perform machine learning algorithms or applications. And that grew up into my first startup foray, which was called Lyric Semiconductor. And we eventually grew up and joined a larger organization, Analog Devices, and put the chips into all kinds of everyday objects and products which probably everyone uses-- phones, and cell phone base stations, and cars, and so forth.
And one of the things I learned from that experience was that hardware and software go hand-in-hand together. Venturing out to start my second company, Gamalon, was really going back to software from hardware, going back to the algorithms and saying, now that we've seen how these initial algorithms compile onto hardware, can we re-envision the design flow and the tool chain for implementing statistical machine learning and deep learning?
Gamalon has-- currently, we're not using any hardware or special hardware. We use the cloud. And we're actually portable across all of the different public clouds. So we run on Google and Microsoft and Amazon clouds, and so forth. And there's a lot in common between the parallelism that happens inside of a specially designed chip and the parallelism and kinds of communication patterns and so forth and compromises that you have to make when you have lots of machines in a data center, in the cloud. So there's many interesting technological relations there. But Gamalon is a cloud SAS company.
And two things about that-- one of the things we do is we-- basically, people can upload database extracts, CSV files, Excel spreadsheets, and so forth, and just dump them all up onto this web page. And our machine will read through it all, and it'll give you back a single, unified, perfect spreadsheet with-- if there was one spreadsheet that initially had some information about a person, and maybe where they lived, and another one had, maybe, an address, and-- I don't know-- something else, like the cost of their home, then you could join those three things together now, even if they were written differently, so even if they were written in colloquial human language, not necessarily written out in machine code.
And what we're really talking about with this new kind of machine learning is a way of bringing stories into deep learning. So it's what we call prior knowledge. So today, the state-of-the-art machine learning doesn't know anything until it meets the data. So it's a blank algorithm. It's essentially a tabula rasa. It has no prior, almost no prior.
And you give it some data, and it learns from that data. It sort of shapes itself. But before you give it some data, the same algorithm could essentially run on audio, video, texts, very similar. Whereas human thought, probably-- and we think the future of machine learning-- lies in the machine actually knowing some stuff. And so we call those stories.
And our machine learns stories from data, but then they're articulated in the system. So we can go in and read the stories it's learned. They're written in Python. And we can see what it's thinking. And when it goes and applies the stories it's learned to the future work that it does, you can have a good sense-- like a human expert with a certain background, you can have a pretty good sense of what kinds of background or prior leanings or ideas it's going to bring to examining a new piece of data.
[MUSIC PLAYING]
-
Interactive transcript
BEN VIGODA: Yeah, so traditionally, there's been two extremes in machine learning and artificial intelligence. Machine learning has tended to not have any stories or prior knowledge baked into it. It just learns from data. And it almost knows almost nothing when it gets started.
On the other end of the spectrum, a lot of the work that was done, for example, at MIT for a long period of time-- the '80s, '90s, early 2000s-- were around artificial intelligence, expert systems, Bayes nets. Systems where humans could program in expertise into the computer. And so those were kind of extremes.
In one extreme, the first extreme, it takes a lot of training examples. If you want to teach a computer what a kitten looks like, then you get a picture of a kitten. You say, that's a kitten. And then another picture. And you say, that's a kitten. And you do that about 10,000 times. Now it knows what a kitten is. If you want to teach it a kazoo, you get 10,000 pictures of kazoo, and you say, kazoo, kazoo, kazoo. So it's a lot of human time to train it.
On the expert systems side, it's also a lot of human time because people were typing in rules. What is the difference between a kitten and a puppy? I don't know. The kitten has whiskers. The kitten has triangular ears. So you have to type in all of these details.
It tends to be very brittle because if something a little bit changes-- there is a different kind of puppy that doesn't look like the average puppy or something like that-- then maybe the rules don't apply. So the rules, you type them in. Takes a lot of time. It's brittle. The training takes a lot of time. And it learns from data, which is great. But you sort of can't tell it anything and you don't know what it's thinking.
So what we've done is sort of find a best of both worlds. And they're sort of soft rules, if you will. The system is learning, essentially, a story about how the data came to be. So in the case of looking at an image, the data on the image came to be because light reflected off of a kitten and hit the aperture of your camera. And the kitten had legs, and a face, and eyes, and so forth.
But they're very general. Typically 50 lines of code. So it's a very simple set of rules. And we find that with just a few rules and, say, three or four training examples-- that's a kitten. That's a kitten. That's a kitten. Generally, kittens have whiskers and they probably have triangular ears. That kind of sweet spot does better than programming 100,000 rules or pointing at 10,000 or or 100,000 kitten pictures. It does better than either of those extremes.
So how does this relate to deep learning, existing deep learning, or rules based systems? Essentially, any deep learning system today or any rules based system could be rewritten in our system. It could be expressed as a special case. So the way to think about it is, if Python is a programming language, or C is a programming language, in a programming language, you could write a program. So you might write a program to, I don't know, spit out the alphabet or something like that.
In Bayesian program learning and Bayesian programming, a deep learning system would be one program, and an expert system would be another program you could write. So what we've really done is come up with a unifying fabric that allows you to express essentially any machine learning probability distribution, any statistical machine learning system, and then sort of combine them into a completely rigorous and unified way with one another and in gradation.
So it's a different way of thinking. Instead of going and grabbing modules, machine learning modules, you just write down a initial story for how your data came to be. And then you push compile, inference compile. And what happens is, under the hood, our cloud examines the story you wrote and figures out how to fit it to the data and whether the story needs to be modified to fit the data, and then tries modifications to fit the story to the data. And it also tells you which data doesn't fit the story.
So one of the things that's most important about our approach is that it is modular, and composable, and has abstraction boundaries the same way regular programming does today. So today, because we've built debuggers, and profilers, and compilers, and regression testers, and all this great machinery that helps programmers program, with regular conventional programming today, you can have a team of 250 programmers, and they can build something like Google Maps. They can each take on-- I can say I'm going to work on the UI. And somebody else can say, I'm going to work on the database back end.
And we don't need to look at every line of code that each other wrote. We can stick them together because we have APIs. We have ways of abstractions for sticking together software. That has not existed in machine learning until today. So basically, the limit for machine learning has been essentially one or two people working on a system, on an algorithm. They have to keep all of the code in their head. When I put a third person, traditionally, on a machine learning project, it would just slow it down and make the outcome riskier and less likely to succeed. So there was really a modularity and a scalability limit before.
One of the things that Bayesian programming does is it makes machine learning like regular programming so that different individuals and different teams can work on different parts and they can be put together. And that's what leads to open source. That's what leads to the sort of software eating the world that is this ability to continually reuse other people's software and incorporate it into new and better systems. And now we're going to be able to do that in machine learning, which I think is the most exciting things about Bayesian programming.
[MUSIC PLAYING]
-
Interactive transcript
BEN VIGODA: We're super grateful, and had a great time working with Stacks, with MIT Stacks. It's been an incredible opportunity to meet the ILP companies and to connect. We still collaborate with Professor Josh Tanenbaum and others at MIT on the academic research side of our company, and then being able to kind of bridge the-- in some ways bridge between gigantic companies in many cases and research still coming out of the lab. That's exactly our sweet spot. That's where that's where we want to be. So for us, this is our sweet spot, where we-- really an exciting opportunity.
We're announcing now our first alpha product. And it's going to be a private alpha, so people hopefully will be eager to join on to clean, prepare and integrate their data completely autonomously. So usually if you have a bunch of different databases and you want to bring them together and to organize them, to duplicate them and sort of make one database, what you have to do is get database integration software or database prep software. Probably you're going to pay it out 10 times as much for the person to operate the software as you did for the software, and you're spend months with the person operating the software, multiple people. And then usually tens or even hundreds of people reviewing the results to make sure that everything is correct and in the new database correctly.
What our system does is essentially takes the human operator, turns that into an artificial intelligence, puts it-- that artificial intelligence in front of the software. You can think of it all as being in one software box, and puts it in the cloud. So it's completely autonomous I.T. operation, taking data from one place to another or multiple places to another and combining it without human intervention. So yeah, we're launching that now with our new private alpha, and for folks who have that kind of a problem coming up in their businesses again and again in an ongoing way, I think this is going to be really exciting. We already have a number of initial customers prior to our launch who we've been working with for the past year.
And it's been awesome. The results that we've been able to achieve for those customers just absolutely fantastic. I'd love to tell you about a couple of case studies that we've done with two of our lead customers. One of them has 700 brick and mortar stores across the US, where they have products on the shelves, and each store has its own sort of computer that's their cash register and their inventory system, and it could be any kind of database.
And what they're doing is dispatching drivers to pick up essentially groceries at these stores and bring them to your house, and they need to link to each of these 700 stores and figure out what products are on the shelves in each of these stores. And it's just incredible how many different ways there is-- there are to write something like Budweiser six pack. You know, Bud Light. So our system goes into each of the 700 databases, automatically reads it all, figures out what's really on the shelves in each of those stores, and then enables them to dispatch drivers in an error free way. When that driver gets to that store, they know that that product is going to be on the shelves.
One of our other use cases is many hundreds of 400 resellers that one of our customers work with. So they're a manufacturing company, and they sell-- they're a wholesaler and they have all these retailers. And they want to know what's going on at each of those retailers. Who are they selling to? So there's databases of all of their end customers. And so we go into all those databases, connect all of that information, line that up with their master contracts list, and we're able to get incredible view, omnichannel view across how products are moving through their distribution channel.
-
Interactive transcript
BEN VIGODA: So you might say software as a service, SaaS You might think of it as machine intelligence as a service, because it's not exactly software that you might use and expect of a GUI. It's really sort of a intelligence in the cloud that you can provide it with data and it'll help you analyze it. And in that sense maybe it's a little bit different than software as a service. It's maybe intelligence as a service.
Five years from now, our dream would be to be essentially the ubiquitous middleware layer for all SaaS software. So one of the things that's happened because of software as a service, because of cloud, it's getting cheaper and easier to make new enterprise software. So I just saw a list of all of the available enterprise SaaS marketing software. There were over 700 applications. Like 700 different marketing apps that you can buy if you're a company to help you do your marketing. So every company buys a different mix of SaaS apps, and every SaaS app stores its data in a different way in some kind of local data store. And it's just this exploding question of, how are you going to get a single global view on what your company is selling, who's selling to, where its inventory is coming from, how much you're paying for it, all those things.
So we see ourselves as essentially an autonomous machine intelligence layer that can just go into any one of those data sources, read it and understand it and pull together for whatever view you need, without any real human intervention. So just a set of pipes, or kind of a utility that just flows information around the enterprise without a lot of effort.
So we've just demonstrated some new scientific results, kind of on the laboratory side of what we do as a startup, where we showed that the more prior knowledge that you pour into a model-- in other words, the richer the story that you tell the computer about the data-- as long as it's the right story. Long as it actually describes the story of the data correctly. The richer, the more info prior knowledge you pour into the system, the faster it learns. And the less labeled data that it needs in order to learn.
And so we call that big model sometimes, as opposed to big data. You don't necessarily need tons of data to train the system. In fact, we show that kind of a general principle is that as we put more and more prior knowledge in, we can reduce the amount of training that the system needs. So you essentially make it smarter.