Luminoso
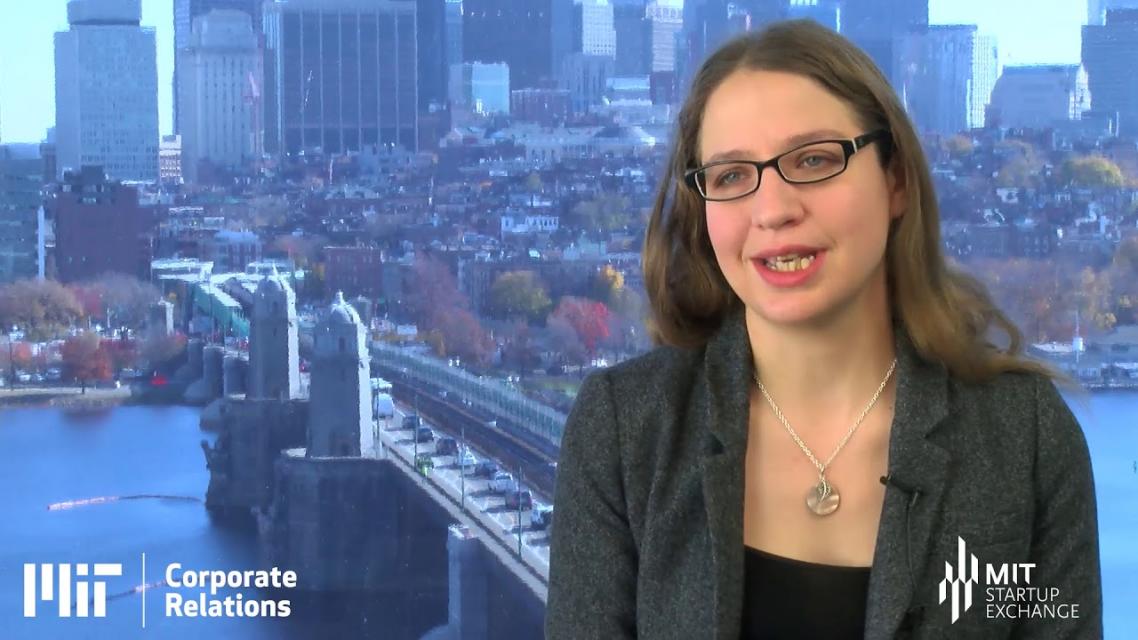
-
Interactive transcript
CATHERINE HAVASI: Hi. I'm Catherine Havasi. I'm co-founder and CEO of Luminoso. We help computers understand people better, and we help companies understand their customers better. At the media lab, I'm a research affiliate, where I run a project called Concept Net that tries to collect all the information that people know and computers don't and put it in a machine readable format.
I think it probably would surprise you that we didn't start out intending to be a text analytics system. We started out in the late '90s actually. I was in Marvin Minsky's Society of Mind group as a [? Europe, ?] and at that point in time, search on the internet had just gotten started. You know, there wasn't really Google as we think of it, and now there's this plethora of search engines. And people had this problem where they would go to the search engine and they would type in something like my cat is sick and they would get nothing that would help him solve their problem. And eventually, how this got solved is that people learned how to search differently and people approached search engines differently.
But at the time, one of the things we were trying was to approach this problem by helping the computer understand more about the context in which people were likely to ask questions so they could do search smarter. So at the time, that meant that we needed to be able to collect them understand all the things that people know and computers don't, and we can think of that as common sense or world knowledge. The technical name for it is actually common sense. But it's things like relationships between physical objects in the world. It's things like what motivates us and what makes us happy. We needed to collect them.
So at the time, we did what we called harnessing the power of bored people on the internet, which would become known as crowdsourcing, and we had a little box and the box said, teach the computer. At the time, I was very enamored with the design work of the little upstart search engine Google, so it had just a little box that looked like Google on a button that said, teach the computer. And so we started collecting knowledge, and we'd check it through each morning. We'd say, yeah, that's true, or yeah, that's not true, and then, eventually, it caught steam and, you know, we couldn't check everything through, so we had to start coming up with automatic ways to check the knowledge and automatic ways to do other things.
As time went on, people were no longer interested in entering knowledge in a box at a computer and not getting anything back from the computer. So from there, we started doing work on what was called games with a purpose. So basically, people would play games, in our case, things like Taboo or, in China and Taiwan, a Tamagotchi pets game, and they would use these games to teach the system while not thinking about it. And towards the end of it, became clear that people were playing Verbosity, which was the Taboo-like game, and they weren't even thinking about teaching the machine. So we got a whole lot of data from that that built on top of our base of data.
More recently, we've used that data to build a system that can automatically look at something like Wikipedia and be able to pull out other bits of information it can use to build out its network. So now we have a network with about 17 million concepts, and it's in all different languages. It's really important to be able to capture all these different sort of cultural nuances and different pieces of information across different cultures.
If we think about it, how we think of a cat and how somebody on the other side of the world thinks of a cat can be very different. The sort of connotations around whether or not it's cute or whether or not it's dirty can be different. And the way we describe what we want out of an experience that we have at a hotel or something like that would be vastly different across different languages, not to mention concepts of flavor or even what time dinner is. So you really need to be able to capture that and add it back in the machine for doing a wide variety of stuff. Text analytics is only one of them. Search is another big one.
And I think it's interesting we've sort of come full circle with search, because now people picked up Siri on their iPhones and they started talking to it like you talk to the search engines in the '90s and they were like, you know, Siri, what-- where can I go to go out to eat that would be-- would fit my mood, and a lot of Siri is done by rules and things like that. And it's the same thing, it has to get-- to actually bring it to the next level, we're going to have to build something that guesses the user's intentions. Or the same thing could happen, and everyone could start trying to talk differently to their phones. I think it remains to be seen.
-
Interactive transcript
CATHERINE HAVASI: Hi. I'm Catherine Havasi. I'm co-founder and CEO of Luminoso. We help computers understand people better, and we help companies understand their customers better. At the media lab, I'm a research affiliate, where I run a project called Concept Net that tries to collect all the information that people know and computers don't and put it in a machine readable format.
I think it probably would surprise you that we didn't start out intending to be a text analytics system. We started out in the late '90s actually. I was in Marvin Minsky's Society of Mind group as a [? Europe, ?] and at that point in time, search on the internet had just gotten started. You know, there wasn't really Google as we think of it, and now there's this plethora of search engines. And people had this problem where they would go to the search engine and they would type in something like my cat is sick and they would get nothing that would help him solve their problem. And eventually, how this got solved is that people learned how to search differently and people approached search engines differently.
But at the time, one of the things we were trying was to approach this problem by helping the computer understand more about the context in which people were likely to ask questions so they could do search smarter. So at the time, that meant that we needed to be able to collect them understand all the things that people know and computers don't, and we can think of that as common sense or world knowledge. The technical name for it is actually common sense. But it's things like relationships between physical objects in the world. It's things like what motivates us and what makes us happy. We needed to collect them.
So at the time, we did what we called harnessing the power of bored people on the internet, which would become known as crowdsourcing, and we had a little box and the box said, teach the computer. At the time, I was very enamored with the design work of the little upstart search engine Google, so it had just a little box that looked like Google on a button that said, teach the computer. And so we started collecting knowledge, and we'd check it through each morning. We'd say, yeah, that's true, or yeah, that's not true, and then, eventually, it caught steam and, you know, we couldn't check everything through, so we had to start coming up with automatic ways to check the knowledge and automatic ways to do other things.
As time went on, people were no longer interested in entering knowledge in a box at a computer and not getting anything back from the computer. So from there, we started doing work on what was called games with a purpose. So basically, people would play games, in our case, things like Taboo or, in China and Taiwan, a Tamagotchi pets game, and they would use these games to teach the system while not thinking about it. And towards the end of it, became clear that people were playing Verbosity, which was the Taboo-like game, and they weren't even thinking about teaching the machine. So we got a whole lot of data from that that built on top of our base of data.
More recently, we've used that data to build a system that can automatically look at something like Wikipedia and be able to pull out other bits of information it can use to build out its network. So now we have a network with about 17 million concepts, and it's in all different languages. It's really important to be able to capture all these different sort of cultural nuances and different pieces of information across different cultures.
If we think about it, how we think of a cat and how somebody on the other side of the world thinks of a cat can be very different. The sort of connotations around whether or not it's cute or whether or not it's dirty can be different. And the way we describe what we want out of an experience that we have at a hotel or something like that would be vastly different across different languages, not to mention concepts of flavor or even what time dinner is. So you really need to be able to capture that and add it back in the machine for doing a wide variety of stuff. Text analytics is only one of them. Search is another big one.
And I think it's interesting we've sort of come full circle with search, because now people picked up Siri on their iPhones and they started talking to it like you talk to the search engines in the '90s and they were like, you know, Siri, what-- where can I go to go out to eat that would be-- would fit my mood, and a lot of Siri is done by rules and things like that. And it's the same thing, it has to get-- to actually bring it to the next level, we're going to have to build something that guesses the user's intentions. Or the same thing could happen, and everyone could start trying to talk differently to their phones. I think it remains to be seen.
-
Interactive transcript
CATHERINE HAVASI: So Luminoso was founded in December of 2010. We had really been working pretty closely with a couple of different companies who were ready to try it out, I think, when it went to hit market, and that was very exciting for us. And one of the biggest things that we figured out right off the bat was that it wasn't just analytics that was important, it was also being able to communicate the results of the computer got that were important. And so one of the early things we did in the company that we hadn't done at MIT was pay attention to visualization and really look into how can we communicate those results. And I think that's also a differentiator besides the technology.
At the time, text analytics in the commercial world really falls into two big categories, one of which is sort of the more statistical techniques that you can think of, and this is things that are driven by how often do words appear next to each other. And it requires a lot of data to get right, because many of the connections that we think of and hold dear are not connections we say all the time. And so in order to even make those connections, you would have to look at a lot of text.
On the flip side of that, there were systems that relied on something called an ontology or a rule set, which is a big, hand-coded essentially aid to the computer to be able to understand the way text worked, but it wasn't adaptive. As the world changed, as the way people talked changed, the ontology didn't keep up, unless you kept it up yourself. And that really was a problem, because consumers are talking in different ways all the time, companies are introducing new SKUs, new other sorts of information. It becomes very difficult to keep up. You're essentially taking the person out of one part of the process and putting it in another part of the process, which isn't really solving your problem.
So Luminoso is a SaaS-based company. We have two products. Our dashboard allows you to take in everything from survey open ends to your chat that your customer has with your representatives on your web page and be able to take it and build out actionable insights on everything, from what features you should improve to be able to make more people be advocates to your product to, you know, which of the items did people buy when they went shopping on Black Friday that were actually the reason they came to your store in the first place. And for the most part, that works with static quantities of data. So you have survey open ends, you have social media from last year, you have form information. There's incredibly rich information probably about your product online somewhere and it's not in social media.
So some of that, I think it's really interesting that there's a lot of text online in places people wouldn't think there are. There are entire forums devoted to shaving, devoted to cars, devoted to how to optimize airline travel, and many people don't look in those places. They look at social, they look on Twitter, and there's a really high quality data there.
So it doesn't really matter where your text comes from, and I think that's one of the strengths of Luminoso. When we're reading text it doesn't matter to us whether or not it's a tweet or whether or not it's English written very properly in the New York Times, we're still going to be able to read it and adapt to it, and I think that's one of the things that our technology is very strong in. When we read and understand things, we learn new words we refine our context dependent definitions of words based on what's going on in the text that we're reading, and our technology does the same thing. It's essentially trying to understand how a particular word is used and seeing if it can make relations or analogies between the way other words were used to be able to guess its meaning.
And thus, it can learn new words, it can learn jargon, it can learn things that words that are now meaning something else. So the carousel on the Amazon Kindle Fire is your home screen with your recommendations, and you know, probably our model based on concept net thought it was horses that went around in a circle. So it needed to be able to learn that, and we needed to be able to build out that capability.
-
Interactive transcript
CATHERINE HAVASI: So I think the idea that we all have world knowledge or common sense that lets us understand the way people think is really important in being able to get under the way we talk in a creative way and the way we talk when we're passionate or excited. We use all these metaphors. We try to say things in new and interesting ways. And one of the reasons we do that, especially online or when we're talking about products, is we want to be interesting. We want to be creative. We want to be the one people listen to.
And so I think it's important to be able to collect that information and add it back in. A company we were talking to had brought up a good example of this problem, which is that someone had called their customer help line and said that their product smelled musty. Now this isn't a product that you want to smell musty, or even that it makes that much sense that it smells musty. But they really need to understand is this something systematic, is there a geographic place where this is happening, is it part of our supply chain, do we have to worry about it, or is this just an isolated incident? And the only way you can do that is look at all your logs and say, how many people called in with the same problem.
Now if you or I were to go through the logs and say, oh, this person had said it smells like an old house. They possibly had the same sort of problem. We would be able to do that really easily. But unless you had pre-trained a computer beforehand to be able to pick up those associations, it wouldn't be able to do it. And that goes into the knowledge that we have that allows us to understand when people are talking about creative things they're probably tying into this knowledge.
So for us, I was running the digital intuition group at the Media Lab and working with my students on this project and other related projects. And I think something that became clear right off the bat was that people were really struggling because there wasn't anything that they could use to work on this, especially with things like developing new products and throughout the product development lifecycle. Being able to understand the text that their consumers generate and the way they talk about things like flavors and sense and colors and textures was something that everybody approached in a really qualitative way, as opposed to a quantitative way. And these companies were really trying to make data driven decisions.
So at the Media Lab we work with our member companies to be able to take technology that we develop and see it and test it in the real world. And we had started doing this with a whole number of companies, looking at everything from how people feel about insurance all the way to how people feel about their shampoo. And it was really showing some very promising results, and I think people really wanted to see it in something that wasn't a research project and something that they could apply to build their companies in a more data driven way.
So we really started out not necessarily just in marketing, although we did start out with a few marketing people. But we also started out doing product development for CPG and food, and I think that's still a big strong area for us. But really right now what we do is at all stages of the product development lifecycle. So our software can help everywhere from being able to figure out whether or not a brand would authentically build a certain kind of product, all the way to figuring out what kind of SKUs drives someone into a retail store. Anywhere you have free text and you would think about it in a qualitative way, and you wish you could think about it in a more data-driven way-- that's where we come in.
[MUSIC PLAYING]
-
Interactive transcript
CATHERINE HAVASI: So Luminoso's second product is called Compass, which allows you to take in streams of text data and be able to process that in real-time to be able to understand things as they evolve, and to be able to get early warning on things that are developing. So the first time we did this was actually for Sony and their digital agency ISO bar when Sony was sponsoring the world cup. So Sony built a second screen experience called One Stadium that allowed fans to be able to watch what was going on during the world cup and the game on their tablet.
And they were also able to generate real-time marketing alongside the information that was going on in social. So we had the opportunity to take in what was, to this point in time, the largest social media event that had ever happened. And I guess it really highlighted for us the difference between big text in an analytical sense and big data in general.
Big text is very variable in its volume. I think that Twitter can be very spiky. If someone scores of gold during the world cup everybody tweets about it, and you don't want to see all of that on your second screen experience. So you need to be able to say who's contributing uniquely and interestingly to the conversation and how much are those topics becoming the topics that people want to know about anyway. You need to be able to react accordingly.
And also, you need to be able to do it in a more dynamic way. New things become important during the world cup that weren't important at the start. So you can't have a person coming up with the keywords. You have to search for-- you need to have the computer go back and do that, and that's something unique to us.
For example, when one player bit another player during the world cup, suddenly the topic of biting became very related to football, to soccer, and that wasn't something we were used to. It wasn't something anybody anticipated coming in. And we need to be able to react very quickly to be able to put the commentary, the thoughts, the jokes that came along with that into the experience.
I think visualizing and explaining the conclusions the computer are coming up with is almost as hard as coming up with the conclusions itself. I think when we talk about analytics and we think about analytics, nobody ever really thinks about communicating the information the computer is coming up with in a way that you can make actionable decisions on it. And I think it's incredibly important and it's incredibly hard.
For our technology, we have essentially a multidimensional vector space that you can use and look at, but you need to portray that in a way that is understandable to someone. And understandable such that you don't even need to think about math or vectors or any of that kind of thing. So for us, we really tapped into the language of visualization, the things that people know how to deal with. People understand word clouds, people understand heat maps. How can we take these more advanced concepts and use them in an analogous way?
I think we're always searching for different ways of visualizing and representing our data in ways that will more clearly convey the opportunities therein to people all over the company, all over the decision making process, all the way from analysts to people who really haven't thought about data before. And how can we take that? How can we build that out? And in the next year I think a lot of the work that we're doing on the main dashboard product will focus in that area.
[MUSIC PLAYING]
-
Interactive transcript
CATHERINE HAVASI: So one other thing, which is Luminoso also has an API that allows you to do things like classification and auto tagging on top of our text analytics platform without working with a GUI. And that allows it to be able to be integrated into different processes and automated decision systems that happen within companies. We can do things like understand if a review comes in what genre of movie the review is likely to talk about, all the way to being able to figure out if someone is a Republican or a Democrat on Twitter.
I think what's next for us is obviously continuing to work on the dashboard. We're working on releasing something that's called the tagging dashboard that really enables users to tag and work with groups of documents, as well as with individual reviews. Being able to take the power of analyzing an individual contribution or many individual contributions and trying to understand how that works together in large groups to make better decisions, and to better search documents to find conceptual answers you're looking for.
So right now, a lot of my research time and a lot of our research time is focused on concept net, which is the model of how people think about the world that Luminoso and hundreds of other people use to be able to bring this commonsense knowledge into computers. And I think for us right now, building what is a huge open data resource and providing it for people is really interesting. Right now our biggest questions and our biggest thoughts are how can we take the work that we've done and move it to languages that are harder to work with than the languages we've looked at.
So right now we're trying to understand can we move this to Arabic? Can we start working with Turkish? Can we look at Malay or Indonesian, and how would we start working in these areas where our traditional collection methods, like games with a purpose, are going to be a little bit more difficult? And being able to say, how can we work across the different dialects of Arabic, things like that? There are all these questions about taking concept net and really bringing it to more of a global reach.
So when we started Luminoso at the Media Lab, one of the things that happened is by the time we actually had started-- the technology was ready for commercialization-- as a research group, we had moved on to other things. So it was very much things that were happening in parallel. Luminoso was working on text analytics, and in the lab we were working on what we thought was going to be the next step for this, which was dialogue-based analytics. Understanding you and I as we talk to each other and understanding how organizations work.
Can we understand organizations from the digital breadcrumbs that they leave behind? Can we see how an organization structures and functions, and can we understand how to connect it better just by the text it creates on a daily basis? And we put a lot of work in to that building the glass infrastructure system, helping to build the glass infrastructure system at the Media Lab. That helped the Media Lab connect with each other and connect with its members based on their interests.
I also had started doing a lot of work on computational creativity and story understanding. So can we build systems that are able to understand and augment a storyteller as they're telling a story? So with students with Dan Novy, we built a system called the [? naratarium, ?] which allows you to tell a story to the computer and it will build interactive visualizations, either based on colors of what you're talking about or based on preset assets in an immersive fashion in the room around you as you're telling a bedtime story. And [? naratarium ?] is a Media Lab project. When you go by the Media Lab feel free to ask to see it.
Right now, Luminoso is focused on market research, marketing, and working with large brands to understand their consumers. But in the future in next year, we'll actually be able to go on premise and build installs within data centers. This will allow us to work with markets that we haven't worked with before such as financial services, pharmaceuticals, and law enforcement.
[MUSIC PLAYING]