Relativity6
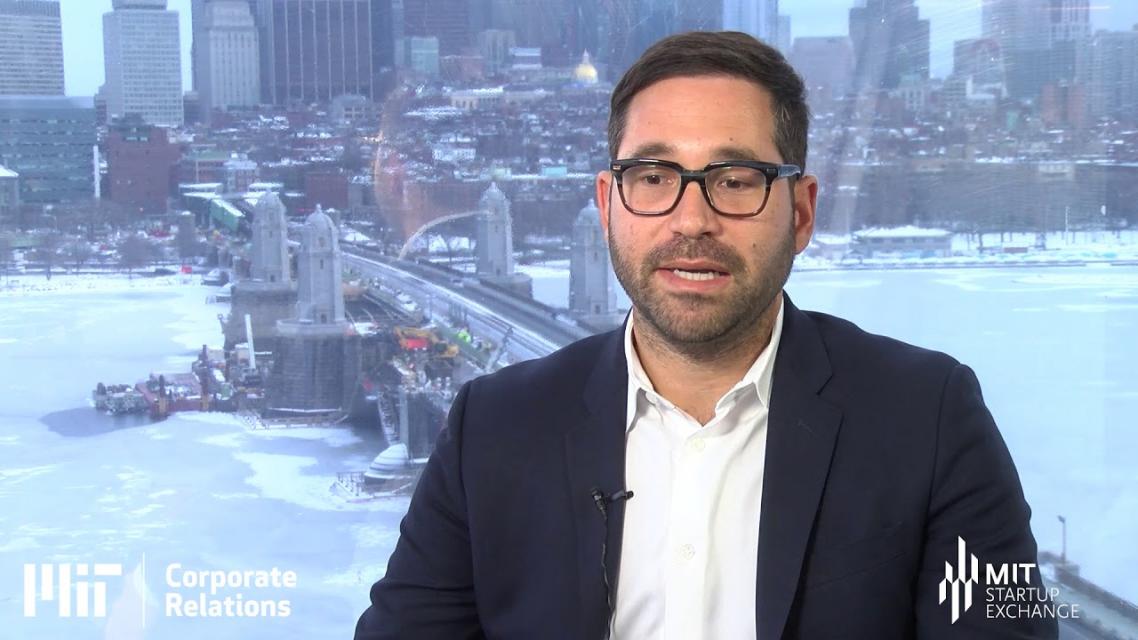
-
Interactive transcript
ALAN RINGWALD: So my name's Alan Ringwald. I'm co-founder and CEO of Relativity6. I started the company out of MIT with my co-founder. The company focuses on reactivating lapsed customers through artificial intelligence.
So the company started very much at MIT. My co-founder and I co-wrote a master's thesis on how unresponsive customers could get reactivated through machine learning. So we spent a better part of a year researching and writing, and our thesis advisor, who also is a Sloan professor, helped us a lot and is actually on our advisory board right now. In terms of the ILP, it's been, again, an instrumental part of Relativity6. We've gotten incredible support from the staff and have already started working with several companies in the network.
While in school, we were also a recipient of the MIT Sandbox Innovation Fund, which was great because it gave us our first money in and gave us a chance to pitch our product for the first time and was the springboard for what eventually became the actual company. In terms of the way we structure the company, I'm the CEO, but my background is in behavioral economics, which is how I contributed to the thesis. I focused more on all outreach sales, strategy, anything that doesn't relate to the actual making or developing of the algorithms. And that's where Abraham steps in, and that's why we're such a great team. So he's the CTO, and he oversees our team of data scientists. And he was the first one to create our initial algorithm that started the whole company.
[MUSIC PLAYING]
-
Interactive transcript
ALAN RINGWALD: So my name's Alan Ringwald. I'm co-founder and CEO of Relativity6. I started the company out of MIT with my co-founder. The company focuses on reactivating lapsed customers through artificial intelligence.
So the company started very much at MIT. My co-founder and I co-wrote a master's thesis on how unresponsive customers could get reactivated through machine learning. So we spent a better part of a year researching and writing, and our thesis advisor, who also is a Sloan professor, helped us a lot and is actually on our advisory board right now. In terms of the ILP, it's been, again, an instrumental part of Relativity6. We've gotten incredible support from the staff and have already started working with several companies in the network.
While in school, we were also a recipient of the MIT Sandbox Innovation Fund, which was great because it gave us our first money in and gave us a chance to pitch our product for the first time and was the springboard for what eventually became the actual company. In terms of the way we structure the company, I'm the CEO, but my background is in behavioral economics, which is how I contributed to the thesis. I focused more on all outreach sales, strategy, anything that doesn't relate to the actual making or developing of the algorithms. And that's where Abraham steps in, and that's why we're such a great team. So he's the CTO, and he oversees our team of data scientists. And he was the first one to create our initial algorithm that started the whole company.
[MUSIC PLAYING]
-
Interactive transcript
ALAN RINGWALD: So machine learning is just incredibly powerful. It's technology that can actually learn from data, without rules-based programming. Meaning you're able to define just an output variable, or result, and let the machine actually decide which variables are dependent on that to actually create that outcome. The implications are just monumental for all kinds of businesses.
The key to machine learning, though, to be successful is making sure you have a defined use case. So that's why I think we've been so successful as we can-- we've been pretty clear about our use case. We work on the lapsed customer problem. We work on looking at customers that have been inactive for a long period of time and understanding how to bring them back. So I think that's where we differentiate ourselves quite a bit is that we've always focused on this. And we feel like we're basically the world leader in this specific use case. And what's great is we can actually prove it with companies over time.
So a lot of companies-- they generally think that people defect for either price, right? So something's too expensive. Or for service, if they had a poor service experience. But we really think that's just the surface.
So what our technology does is look for the hidden variables that aren't necessarily so obvious. Maybe someone's tastes changed or maybe there's new competitor in the market. So that's another piece of our technology is actually going in, looking at the observable variables that are there, but using that to help uncover the hidden variables.
Typically companies over invest in acquisition, and even some retention strategies, because, again, it's a very broad definition of how they go attack that. Because we work on a very individual customer level, we're able to tell companies specifically to email this cohort, or call this other cohort, or send this other group of people a catalog instead, or maybe re target another group, right. So companies are extremely efficient in the way that they go and reacquire these companies based on our insights.
So the companies that have participated in the pilots have actually ranged greatly, right. So we have companies that have millions of customers, literally, 50 million plus customers. But we also have companies that have 1,000 customers. But it's OK. What actually matters is that they've collected enough data for us to analyze. And what that means is that we have at least 18 months back of data because the way our algorithms work is they'll predict the past and see how well it does. And then once it feels confident enough about its past predictions is when we can feel good about predicting future behaviors.
The technology behind Relativity6 is quite streamlined at this point. The way it works is initially we ask for purely behavioral data from a company. We don't want names. We don't want emails. Nothing personal. We just need a unique customer identifier. Because what we're doing is we're analyzing internal past purchase behaviors.
So once we get the raw data from a customer, we actually go ahead and transform that data to make it useful for our machine learning algorithms. We then take those out-- we then take that data, churn it through our algorithms, and come out with initial predictions of not only who's going to make a purchase in the next month that hasn't purchased in a while, but also which product or service they're most likely to want to buy. We're also able to predict which channel they're most likely to respond to, whether that's email, phone, catalog.
So then once we have those predictions, companies actually go ahead and use our suggestions. And we get to see what happened from those predictions. And whether we're right or wrong the model retrains itself. It's the beauty of machine learning. It learns whether it was accurate or not. And then is able to retrain and be more accurate the next time around. And that's the process that repeats itself until we achieve that 80% accuracy rate.
In terms of ideal customer, the truth is that it's any organization that's been around for over two, three years that has a lot of customers. It has some that haven't purchased in a long period of time, that has behavioral purchase data, right. What we're looking for is any indicators of actual purchases. So retailers are great because they have so much information. Financial institutions are also great. Insurance companies are also great. But that's not it, right. So literally any organization that's kept their data and has enough customers for us to be able to analyze.
We take data privacy extremely seriously. We even have a couple cybersecurity PhDs on staff. But we-- again, we don't want names. We don't want emails. We don't want credit card numbers. It doesn't even help our model. And we don't want it. We want behavioral data from your customers. So all we need is a unique customer identifier and that's good to go. So that's really helped in terms of working with financial institutions, insurance agencies, hospitals, that kind of thing.
So our models actually work really well with political organizations and nonprofits and universities, in terms of gifting and donations. It's the same-- almost the same exact concept of identifying people that have taken that action before, haven't in a while, and really figuring out the best time and best channel to ask again.
[MUSIC PLAYING]
-
Interactive transcript
ALAN RINGWALD: So the question of the lapsed customer-- it's really interesting. It's what we spent our time writing our thesis on. A lot of companies have definitions for what they consider to be an inactive or a lapsed or a lost customer. We've heard anything from as low as six months to three, four, five years.
What we found, though, is that those decisions aren't necessarily data driven. So a lot of it relies on third party data or survey data or just anecdotal evidence from the company. And what we found is that each individual person actually has their own definition of lapsed, right. So instead of giving a broad cut of saying our customer base, after 12 months of not buying, we consider them lapsed, actually it's individual. And that's one of the algorithms that we built is actually identifying individual definition of lapsed.
The reality is a lot of companies, after they consider someone lapsed, they won't really put many resources towards bringing them back, right? So maybe they'll send an email once in a while. But generally they consider them lost customers.
So what we're doing is actually creating a lot of incremental revenue for the companies because instead of going out and spending all their budget on acquiring new customers, they're going after people that have already had an experience with that company, whether they use a service or bought their product. So they're actually knowing when to ask them to come back and what to offer them is huge because, you know, with one touch they can actually bring someone back who's been gone. And that person who comes back, actually, statistically, is more profitable than a new customer.
Lapsed customers actually have a lot more data than new customers, right, because a new customer is still a prospect. It's still somebody that hasn't interacted with your specific company yet. What's amazing about lapsed customers is they've already had that experience, right? It's almost like an elevated version of re targeting. It's not just somebody visited your website, they bought. And you can see all the actions associated with that. And that's the basis for our algorithms. And that's why they're so powerful.
[MUSIC PLAYING]
-
Interactive transcript
ALAN RINGWALD: The ILP was generous enough to invite me to pitch at the Consumer Dynamic Conference. It was awesome. I've been in the Media Lab a million times and that was my first time presenting. So that was super cool.
I got to say it was a really, really big moment for the company because we were able to present in front of a lot of people and also a lot of companies that were interested in what we were doing. And from there, we engaged with numerous companies and are now working with them. So it was a really great springboard for commercializing the company.
Yeah, things have changed a lot in a short period of time. We've only been in business for nine months, even though we've been working on the idea for a while, been out in the market for nine months. And at first, we were just so excited to get a data set. That was a big deal for us. It's a big deal for any data scientist.
But now, it's just a totally different ballgame. We have many companies that we're engaged with. We have a lot of results back. Our business model is pay by performance so we kind of put ourselves to that standard of being that good. And luckily, we've achieved a lot of what we set out to. And we have a lot more to go in terms of really decoding reasons for defection, which is kind of the next frontier for us. But in terms of our core offering, we found a lot of success early on and just really excited for the future.
I think the word innovation is tossed around a lot. And for us, I think that innovation is taking what we've learned in school, which is a great and useful, but it's like an echo chamber, right? So it's nice when you're sitting in a room and talking about it. When it becomes real and interesting is when you take it out into the field and test it with real companies and put yourself to the standard of being successful. Because there is no safety net and you have to continue to-- you have to create value, to the point where someone's willing to pay you for it. And I think that that's where innovation actually starts is when you can get your hands dirty and start working and start delivering true results.
[MUSIC PLAYING]
-
Interactive transcript
ALAN RINGWALD: Next is really uncovering a lot of the hidden variables out there in terms of understanding why people defect in the first place. It's very similar to polling data or kind of like the election results lately that people are just aren't incentivized to be truthful. So the way that companies go about understanding reasons for defection now are they ask, they'll give you a survey. But the truth is that most people are not incentivized to be honest.
So what we want to do is take all available data and start coming up with correct hypotheses as to why people defected and then testing them in the field to validate the predictions. So that's the next frontier is being able to automate the process of a predicting why somebody defected, like really defected not just what they said, and testing it out in the field and validating it and doing that over and over in an automated way.
[MUSIC PLAYING]