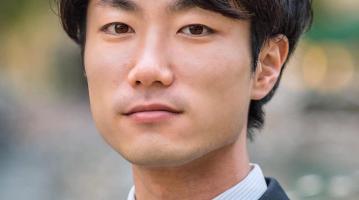
10.10.23-Showcase-Seoul-Themis_AI
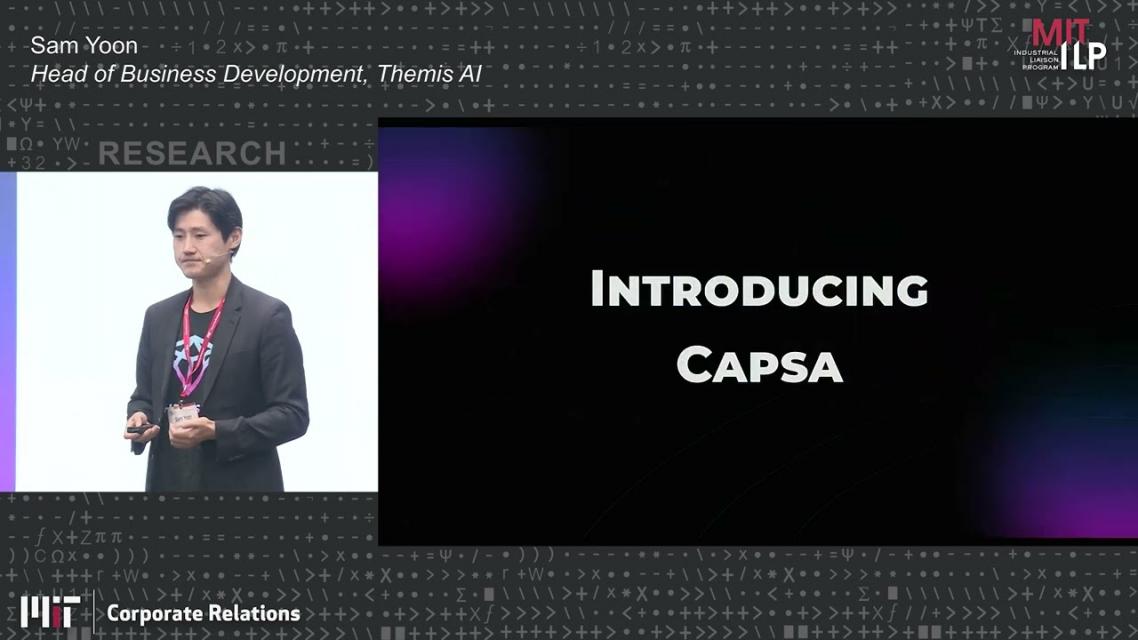
-
Video details
Startup Lightening Talk
-
Interactive transcript
SAM YOON: [SPEAKING KOREAN]
So we can see that AI is being used in many parts of our lives but is still struggling to penetrate the high-risk real value areas. That is a problem that Themis AI is trying to serve. My name is Sam Yoon, head of business development at Themis AI. And we are a team of MIT and Harvard-trained engineers, lawyers, and business professionals that are trying to solve that problem.
After more than five years of research at MIT's renowned CSAIL lab, we have identified numerous challenges of deploying generative AI technologies in a way that people can use in valuable areas like self-driving cars, health care, or finances. We are very proud to bring the technology that we bought from MIT to Korea because we know that Korea is trying to become a leader in AI deployment. And a key part of that process is making sure that we can use AI safely.
For example, we have been working closely with Korean regulators around how to deploy technologies that come from around the world, such as MIT, to make sure that we can use AI in a safe way so that we can ensure public trust. I'd like to show you a few examples of how technology can be used.
So we've been working with an autonomous vehicle provider in the US to use our technology to make sure that they can enable high trust when they deploy their vehicles onto the road. And we have proved in a lab environment that by nearly 90%, we have increased the success rate for this company.
Another typical example of where we use our technology is in generative AI applications. So many of our presenters today talked about this concept called hallucination, where basically, when images or content is generated, like ChatGPT, for example, we don't exactly know where it is going wrong.
You can use our technology to wrap any existing machine learning model that you have. In this case, we've been working with a leading image-generating company in the US called Stable Diffusion. And we could identify where in their particular output it went wrong.
So you can see that the left-hand side image is a hand image that looks a little bit weird. Obviously, you won't be able to use that in important situations-- maybe in a school assignment. But when you're trying to use it in any kind of commercial applications, you can see the clear problem.
With just a few lines of code, we can wrap any existing infrastructure that you have to identify what part of the image is going wrong. And then our clients use this to either retrain the model or let humans intervene to make sure that whatever output goes out to reality they can control the quality of.
The technology that enables this is Capsa. Our beauty is that we differentiate from other uncertainty metrics by deploying this very heavy R&D in just a matter of code-- few lines of code using a wrapper. So a lot of the leading AI labs that we've been working with, instead of investing a few weeks, sometimes even months of our AI engineers' time to deploy this uncertainty ecosystem, they can tap into our existing libraries, where we've already worked with regulators to make sure that it is of high quality.
This is the example that I mentioned before. And the key part here is that even with a very sophisticated model, like Stable Diffusion, approximately 1 billion parameters, our technology still works at that level. And our algorithm is both model and data agnostic. So today, I've showed you a few image use cases. But it also works with language models, audio models as well.
Another interesting use case that has been worked by a few of our clients is to have higher quality prompt engineering. So prompt engineering is a key part of the user's experience when it comes to generative AI. As you can see, on the left-hand side, we inputted "closeup woman's hands, 4K." And that was the output that we got.
Because we can identify the quality of different images, we can use that data to therefore iterate on prompts as well. So over time, by using a more higher fidelity of inputs, you can also generate higher quality outputs as well. So when we start using these synthetic data to train more sophisticated AI algorithms, our technology has been used to increase the quality of those data points.
Our technology can also be used to make LLMs more certainty aware as well. In a nutshell, Capsa provides a very important technology that is needed as we move from where AI is being used in search results, product algorithms. What we want in the future is for AI to be deployed in hospitals, on the roads, in many more high-risk areas. And that is where trustworthiness becomes very important.
We have already partnered with many Fortune 500 companies to deploy our MIT research to make their machine learning models more aware. And last year, we raised approximately $3 million of investment to launch into the Asian market as well. So we are very excited to talk with different partners in Korea. I myself speak Korean, which is very convenient and perhaps why we chose this market. But we're very excited to work with you all.
Right now, we've been working with companies in these types of industries. One example is a big tech company that is trying to use LLMs for health care diagnoses. And you can imagine why trustworthy outputs is super important in that situation.
So we have Themis AI. We have a booth outside. We'd love to talk to you. And thank you very much for your time today.
-
Video details
Startup Lightening Talk
-
Interactive transcript
SAM YOON: [SPEAKING KOREAN]
So we can see that AI is being used in many parts of our lives but is still struggling to penetrate the high-risk real value areas. That is a problem that Themis AI is trying to serve. My name is Sam Yoon, head of business development at Themis AI. And we are a team of MIT and Harvard-trained engineers, lawyers, and business professionals that are trying to solve that problem.
After more than five years of research at MIT's renowned CSAIL lab, we have identified numerous challenges of deploying generative AI technologies in a way that people can use in valuable areas like self-driving cars, health care, or finances. We are very proud to bring the technology that we bought from MIT to Korea because we know that Korea is trying to become a leader in AI deployment. And a key part of that process is making sure that we can use AI safely.
For example, we have been working closely with Korean regulators around how to deploy technologies that come from around the world, such as MIT, to make sure that we can use AI in a safe way so that we can ensure public trust. I'd like to show you a few examples of how technology can be used.
So we've been working with an autonomous vehicle provider in the US to use our technology to make sure that they can enable high trust when they deploy their vehicles onto the road. And we have proved in a lab environment that by nearly 90%, we have increased the success rate for this company.
Another typical example of where we use our technology is in generative AI applications. So many of our presenters today talked about this concept called hallucination, where basically, when images or content is generated, like ChatGPT, for example, we don't exactly know where it is going wrong.
You can use our technology to wrap any existing machine learning model that you have. In this case, we've been working with a leading image-generating company in the US called Stable Diffusion. And we could identify where in their particular output it went wrong.
So you can see that the left-hand side image is a hand image that looks a little bit weird. Obviously, you won't be able to use that in important situations-- maybe in a school assignment. But when you're trying to use it in any kind of commercial applications, you can see the clear problem.
With just a few lines of code, we can wrap any existing infrastructure that you have to identify what part of the image is going wrong. And then our clients use this to either retrain the model or let humans intervene to make sure that whatever output goes out to reality they can control the quality of.
The technology that enables this is Capsa. Our beauty is that we differentiate from other uncertainty metrics by deploying this very heavy R&D in just a matter of code-- few lines of code using a wrapper. So a lot of the leading AI labs that we've been working with, instead of investing a few weeks, sometimes even months of our AI engineers' time to deploy this uncertainty ecosystem, they can tap into our existing libraries, where we've already worked with regulators to make sure that it is of high quality.
This is the example that I mentioned before. And the key part here is that even with a very sophisticated model, like Stable Diffusion, approximately 1 billion parameters, our technology still works at that level. And our algorithm is both model and data agnostic. So today, I've showed you a few image use cases. But it also works with language models, audio models as well.
Another interesting use case that has been worked by a few of our clients is to have higher quality prompt engineering. So prompt engineering is a key part of the user's experience when it comes to generative AI. As you can see, on the left-hand side, we inputted "closeup woman's hands, 4K." And that was the output that we got.
Because we can identify the quality of different images, we can use that data to therefore iterate on prompts as well. So over time, by using a more higher fidelity of inputs, you can also generate higher quality outputs as well. So when we start using these synthetic data to train more sophisticated AI algorithms, our technology has been used to increase the quality of those data points.
Our technology can also be used to make LLMs more certainty aware as well. In a nutshell, Capsa provides a very important technology that is needed as we move from where AI is being used in search results, product algorithms. What we want in the future is for AI to be deployed in hospitals, on the roads, in many more high-risk areas. And that is where trustworthiness becomes very important.
We have already partnered with many Fortune 500 companies to deploy our MIT research to make their machine learning models more aware. And last year, we raised approximately $3 million of investment to launch into the Asian market as well. So we are very excited to talk with different partners in Korea. I myself speak Korean, which is very convenient and perhaps why we chose this market. But we're very excited to work with you all.
Right now, we've been working with companies in these types of industries. One example is a big tech company that is trying to use LLMs for health care diagnoses. And you can imagine why trustworthy outputs is super important in that situation.
So we have Themis AI. We have a booth outside. We'd love to talk to you. And thank you very much for your time today.