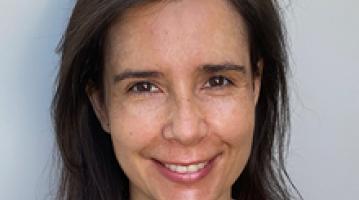
2.28-29.24-Ethics-Nara-Logics

-
Video details
Nara Logics, Artificial Intelligence for the Real World
-
Interactive transcript
SNEJANA SHEGHEVA: Hello there. OK. I'm Snejana. It's pronounced exactly as it's written. And I'm originally from Moldova, which was mentioned this morning, and I'm very excited about that.
I write code generally. I like writing code. But today, I'm here in front of you, talking about explainable AI. My background is in math, in the cognitive AI. But our connection to MIT is through Dr. Nathan Wilson, who has studied at Department of Brain and Cognitive Sciences and done some groundbreaking research.
OK. So at Nara Logics, what do we do? We are a Boston-based company. And we specialize in crafting intelligent advisors across different sectors, sectors such as finance, health care, and I have a personal bias for space and defense. And our focus in differentiation. Big differentiation in explainable AI is what is what I'm going to be talking about today here.
OK. So what is the problem? The problem is that only about 10% to 50%, depending on what kind of AI is used, is being deployed to production. And that's obviously a problem.
So why is that a problem? That's a problem because-- I identified about three main reasons-- that there's a lacking adaptability. Different users have different expertise levels and different needs. Therefore, AI has to make sense to different audiences.
Another one is poor handling and evolving context. I've been there. Done that. There's a model that's six months old. And suddenly, we spend hours and days and weeks debugging why it's not working anymore.
And finally, AI, it seems to be ineffective in highly dimensional data. That is a very hard problem when you receive data from multiple, multiple different sources and build a model on that and, more importantly, explain it back to the user so users understand what they need to do and what kind of action they need to take. Right?
So all of these problems, what they end up? They end up confusing the users. So they don't understand the rationale behind the AI solutions. So we are here with our process, what we call Explainability 360.
So our fundamental-- moving my head a lot. I'm sorry. At Nara Logics, we've asked the fundamental question, explainable to whom? Who has to understand our solution? Who has to make sense of that? And based on that, we tailor our explainability to multiple different roles.
Our intelligent advisors can be activated based on a role, based on what a user from different expert level, whether they are operators or they are the high-level managers, what they need to know. So we tailor our transparency to different audiences.
Our mission is very critical. And so what we want to bring into the table? We want to build bidirectional understanding between machines and people. It's not just about explaining the algorithms anymore. It's about having a conversation, having a conversation between people and machines. And that conversation has to be about sharing knowledge and understanding and insights.
All of this together is what brings trust, safety, clarity, and fairness. That brings understanding. And that understanding is what is important to be able to deploy something to production.
All right. So I'm going to focus on this one use case that we have that I have personal bias for. So we have satellite, satellite maneuver analysis. Imagine they have a satellite orbiting the Earth. What information can we collect about that? What is important?
At first, there can be some very straightforward features that you can collect from that. But what is hidden is a wealth of information that relates to interaction of that satellite with other satellites. So at the core of our explainable solution is the concept from category theory. And that concept is Yoneda lemma.
What that tells us is in order to understand an object, you have to see how that object relates to others through different morphisms. These morphisms is what captures the structure. So it's not important what an object or a process is.
Well, it's important. But what's equally important is also what this project, what this process, what this object is not. And what's important is in what way it's not, in what way it's different from the expected processes, in what way the other processes do not resemble the process we're observing. That's where the Yoneda lemma is. If you want to talk more about that, I'm happy to geek about the math part of it.
All righty. Here we go. And I'm going to skip that one. And that's our partnership ask, is about the defense and intelligence, financial services, and health care. And come talk to us because we'd like to move from-- we'd like to move to data to action. And the last thing is the future requires nothing less. So let's talk.
-
Video details
Nara Logics, Artificial Intelligence for the Real World
-
Interactive transcript
SNEJANA SHEGHEVA: Hello there. OK. I'm Snejana. It's pronounced exactly as it's written. And I'm originally from Moldova, which was mentioned this morning, and I'm very excited about that.
I write code generally. I like writing code. But today, I'm here in front of you, talking about explainable AI. My background is in math, in the cognitive AI. But our connection to MIT is through Dr. Nathan Wilson, who has studied at Department of Brain and Cognitive Sciences and done some groundbreaking research.
OK. So at Nara Logics, what do we do? We are a Boston-based company. And we specialize in crafting intelligent advisors across different sectors, sectors such as finance, health care, and I have a personal bias for space and defense. And our focus in differentiation. Big differentiation in explainable AI is what is what I'm going to be talking about today here.
OK. So what is the problem? The problem is that only about 10% to 50%, depending on what kind of AI is used, is being deployed to production. And that's obviously a problem.
So why is that a problem? That's a problem because-- I identified about three main reasons-- that there's a lacking adaptability. Different users have different expertise levels and different needs. Therefore, AI has to make sense to different audiences.
Another one is poor handling and evolving context. I've been there. Done that. There's a model that's six months old. And suddenly, we spend hours and days and weeks debugging why it's not working anymore.
And finally, AI, it seems to be ineffective in highly dimensional data. That is a very hard problem when you receive data from multiple, multiple different sources and build a model on that and, more importantly, explain it back to the user so users understand what they need to do and what kind of action they need to take. Right?
So all of these problems, what they end up? They end up confusing the users. So they don't understand the rationale behind the AI solutions. So we are here with our process, what we call Explainability 360.
So our fundamental-- moving my head a lot. I'm sorry. At Nara Logics, we've asked the fundamental question, explainable to whom? Who has to understand our solution? Who has to make sense of that? And based on that, we tailor our explainability to multiple different roles.
Our intelligent advisors can be activated based on a role, based on what a user from different expert level, whether they are operators or they are the high-level managers, what they need to know. So we tailor our transparency to different audiences.
Our mission is very critical. And so what we want to bring into the table? We want to build bidirectional understanding between machines and people. It's not just about explaining the algorithms anymore. It's about having a conversation, having a conversation between people and machines. And that conversation has to be about sharing knowledge and understanding and insights.
All of this together is what brings trust, safety, clarity, and fairness. That brings understanding. And that understanding is what is important to be able to deploy something to production.
All right. So I'm going to focus on this one use case that we have that I have personal bias for. So we have satellite, satellite maneuver analysis. Imagine they have a satellite orbiting the Earth. What information can we collect about that? What is important?
At first, there can be some very straightforward features that you can collect from that. But what is hidden is a wealth of information that relates to interaction of that satellite with other satellites. So at the core of our explainable solution is the concept from category theory. And that concept is Yoneda lemma.
What that tells us is in order to understand an object, you have to see how that object relates to others through different morphisms. These morphisms is what captures the structure. So it's not important what an object or a process is.
Well, it's important. But what's equally important is also what this project, what this process, what this object is not. And what's important is in what way it's not, in what way it's different from the expected processes, in what way the other processes do not resemble the process we're observing. That's where the Yoneda lemma is. If you want to talk more about that, I'm happy to geek about the math part of it.
All righty. Here we go. And I'm going to skip that one. And that's our partnership ask, is about the defense and intelligence, financial services, and health care. And come talk to us because we'd like to move from-- we'd like to move to data to action. And the last thing is the future requires nothing less. So let's talk.