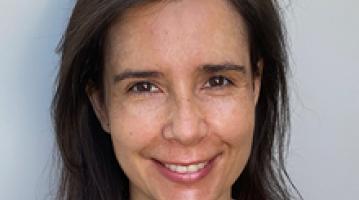
2.28-29.24-Ethics-Sparkdit

-
Video details
Right is Might
-
Interactive transcript
FADI MICAELIAN: And thank you for being here. And thank you for ILP for inviting us to speak today. I'm going to talk to you about augmenting AI with decision intelligence and how it's serving our customers. I'm going to give you that with two use cases. The first one is the airplane swapping. The airline industry is marred by delays. The extent of the problem is $30 billion. And that's anything from pouring your coffee on the carpet to an engine exploding.
Airlines, and particularly airplane manufacturer, if the plane is not in the air, they are losing money. So they tried really hard to solve this problem. And they used this operational research-- did not work. They used AI. And with the AI, they are still having a lot of problems. So they came to us, and they asked us, can we use-- can you solve this problem with augmenting AI with decision intelligence?
And the reason why AI did not work is because there was four key problems. One is data volume. You can see here the difference between the Google number of search per second, 100,000, and the number of flights delayed per second, 0.01. That gives you a sense-- for them, the data volume is not there. The second one is data quality. You have to have uniform data quality in order to go to AIML. Otherwise, junk in is junk out.
And the problem of that is that you don't have that data. When someone comes in and fix a gauge, for example, it might be fixing really the problem. It might be really in the-- somewhere else. And that might take a lot longer. How many times you've been on an airplane that says it's half an hour delayed and became two hours, then it became three hours delays.
The third problem is causality. AI is a black box. It doesn't solve-- and it doesn't tell you why it classifies something in a certain way. They need to understand what was the problem. And the last thing, goal setting is completely foreign for AI. AI does not understand this concept of goal setting.
So we solve it by incorporating decision intelligence. We mimic the way the brain works. We say, OK, how do we think when we solve a problem? Well, the first thing is we have to understand what are the criteria that are taking into consideration to make a decision. And for that, we can use expert knowledge, or we can do it at scale with generative AI.
The second thing is we need to understand how we think about those criteria. What's the criteria behavior? And for that, we use also ML and AI and data modeling. And we associate those things with a set of utility curves. Finally, and that's the key difference, when we make a decision, we're using tradeoffs. And subconsciously, we make tradeoffs. And we are the only company that uses tradeoffs.
Tradeoffs is key to our success. Tradeoff is key to our customer success. And they can be done either explicitly by bringing back the user in the middle of AI, or they can be done implicitly or inferred by using reverse propagation techniques.
So in the case of-- in the example of the airline, what we had, we had initially considerations such as size, capacity of the airplane when you're trying to swap airplanes, repair time, unknown, that can vary quite a bit, cascading delays, how many other problems you have, hubs, crew, and other problems. That gets converted into three-- thanks to generative AI, into three main problems.
It says you have customer satisfaction, cost reduction, and revenue optimization. And then with generative AI, we generate the best function that mimics those three criteria. But more importantly, we introduce the tradeoffs. And tradeoffs allow you to say what's important. So around the end of the quarter, you're saying, maybe I'm short on revenue. Revenue becomes more important. And you can do that dynamically because now you have access to the tradeoffs as a user. You bring back the user at the center of AI.
So the value proposition was that we reduced by 20% the operation efficiency. The second use case is the use case of e-commerce. So in e-commerce, what you have, you go to a website like Nike, they ask you which color do you want. And you can say, I like blue, and you can say I like pink. But the way you think is, I love blue, but I think the pink is 50% less. That you cannot do without tradeoffs.
The second thing is they ask you pick the technology. And that's, by the way, the core criteria that they use to select the cleats. And the solution they have is Flyknit, Lunarlon, and FlyEase, which means nothing to anyone. In our technology, what we do, we ask you, what's your soccer style? How do you play soccer? Is it speed? Is it dribble? Is it shot? Is it precision? And you trade off those things. You see what's important more than others. And that's how we get much better and much more personalized results.
We get 2x conversion rate, 20% revenue increase, and no private data. We don't touch private data. We're looking for partners in the vertical sector of e-commerce, in health care, where we're getting a lot of success and traction in security and government and financial services. Thank you for attention.
-
Video details
Right is Might
-
Interactive transcript
FADI MICAELIAN: And thank you for being here. And thank you for ILP for inviting us to speak today. I'm going to talk to you about augmenting AI with decision intelligence and how it's serving our customers. I'm going to give you that with two use cases. The first one is the airplane swapping. The airline industry is marred by delays. The extent of the problem is $30 billion. And that's anything from pouring your coffee on the carpet to an engine exploding.
Airlines, and particularly airplane manufacturer, if the plane is not in the air, they are losing money. So they tried really hard to solve this problem. And they used this operational research-- did not work. They used AI. And with the AI, they are still having a lot of problems. So they came to us, and they asked us, can we use-- can you solve this problem with augmenting AI with decision intelligence?
And the reason why AI did not work is because there was four key problems. One is data volume. You can see here the difference between the Google number of search per second, 100,000, and the number of flights delayed per second, 0.01. That gives you a sense-- for them, the data volume is not there. The second one is data quality. You have to have uniform data quality in order to go to AIML. Otherwise, junk in is junk out.
And the problem of that is that you don't have that data. When someone comes in and fix a gauge, for example, it might be fixing really the problem. It might be really in the-- somewhere else. And that might take a lot longer. How many times you've been on an airplane that says it's half an hour delayed and became two hours, then it became three hours delays.
The third problem is causality. AI is a black box. It doesn't solve-- and it doesn't tell you why it classifies something in a certain way. They need to understand what was the problem. And the last thing, goal setting is completely foreign for AI. AI does not understand this concept of goal setting.
So we solve it by incorporating decision intelligence. We mimic the way the brain works. We say, OK, how do we think when we solve a problem? Well, the first thing is we have to understand what are the criteria that are taking into consideration to make a decision. And for that, we can use expert knowledge, or we can do it at scale with generative AI.
The second thing is we need to understand how we think about those criteria. What's the criteria behavior? And for that, we use also ML and AI and data modeling. And we associate those things with a set of utility curves. Finally, and that's the key difference, when we make a decision, we're using tradeoffs. And subconsciously, we make tradeoffs. And we are the only company that uses tradeoffs.
Tradeoffs is key to our success. Tradeoff is key to our customer success. And they can be done either explicitly by bringing back the user in the middle of AI, or they can be done implicitly or inferred by using reverse propagation techniques.
So in the case of-- in the example of the airline, what we had, we had initially considerations such as size, capacity of the airplane when you're trying to swap airplanes, repair time, unknown, that can vary quite a bit, cascading delays, how many other problems you have, hubs, crew, and other problems. That gets converted into three-- thanks to generative AI, into three main problems.
It says you have customer satisfaction, cost reduction, and revenue optimization. And then with generative AI, we generate the best function that mimics those three criteria. But more importantly, we introduce the tradeoffs. And tradeoffs allow you to say what's important. So around the end of the quarter, you're saying, maybe I'm short on revenue. Revenue becomes more important. And you can do that dynamically because now you have access to the tradeoffs as a user. You bring back the user at the center of AI.
So the value proposition was that we reduced by 20% the operation efficiency. The second use case is the use case of e-commerce. So in e-commerce, what you have, you go to a website like Nike, they ask you which color do you want. And you can say, I like blue, and you can say I like pink. But the way you think is, I love blue, but I think the pink is 50% less. That you cannot do without tradeoffs.
The second thing is they ask you pick the technology. And that's, by the way, the core criteria that they use to select the cleats. And the solution they have is Flyknit, Lunarlon, and FlyEase, which means nothing to anyone. In our technology, what we do, we ask you, what's your soccer style? How do you play soccer? Is it speed? Is it dribble? Is it shot? Is it precision? And you trade off those things. You see what's important more than others. And that's how we get much better and much more personalized results.
We get 2x conversion rate, 20% revenue increase, and no private data. We don't touch private data. We're looking for partners in the vertical sector of e-commerce, in health care, where we're getting a lot of success and traction in security and government and financial services. Thank you for attention.